With an incredible 2.5 quintillion bytes of data generated daily, data scientists are busier than ever. The more information we have, the more we can do with it. And data science provides us with methods to make use of this data. So, while you search for the definition of “quintillion,” Google is probably learning that you have this knowledge gap.
But understanding and interpreting data is just the final stage of a long journey, as the information goes from its raw format to fancy analytical boards. There’s a whole dedicated ecosystem, so along with data scientists who mainly work with data processing algorithms, there are data engineers who develop and manage the end-to-end data infrastructure.
The 2020 Dice Tech Job Report named data engineers the fastest-growing position in the tech industry. Their 2022 Report continues to observe the increasing demand for these specialists with 42.2 percent year-over-year growth in the number of open positions.
In this article, we’ll explain what a data engineer is and talk about their scope of responsibilities and skill set. We’ll also describe how data engineers are different from other related roles and mention the required education and background for those who pursue a career in this field.
A data engineer is one of the key technical roles needed for successful master data management implementation. Learn more in our dedicated article.
What is a data engineer?
We’ll go from the big picture to the details. Data engineering is a part of data science, a broad term that encompasses many fields of knowledge related to working with data. At its core, data science is all about getting data for analysis to produce meaningful and useful insights. The data can be further applied to provide value for machine learning, data stream analysis, business intelligence, or any other type of analytics.
While data science and data scientists in particular are concerned with exploring data, extracting insights from it, and building machine learning algorithms, data engineering is about building and maintaining pipelines to provide ML algorithms with quality data and managing data infrastructure in general.
So a data engineer is an engineering role within a data science team or any data-related project that involves creating and managing the technological part of data infrastructure.
Check our video on data engineering to learn more.
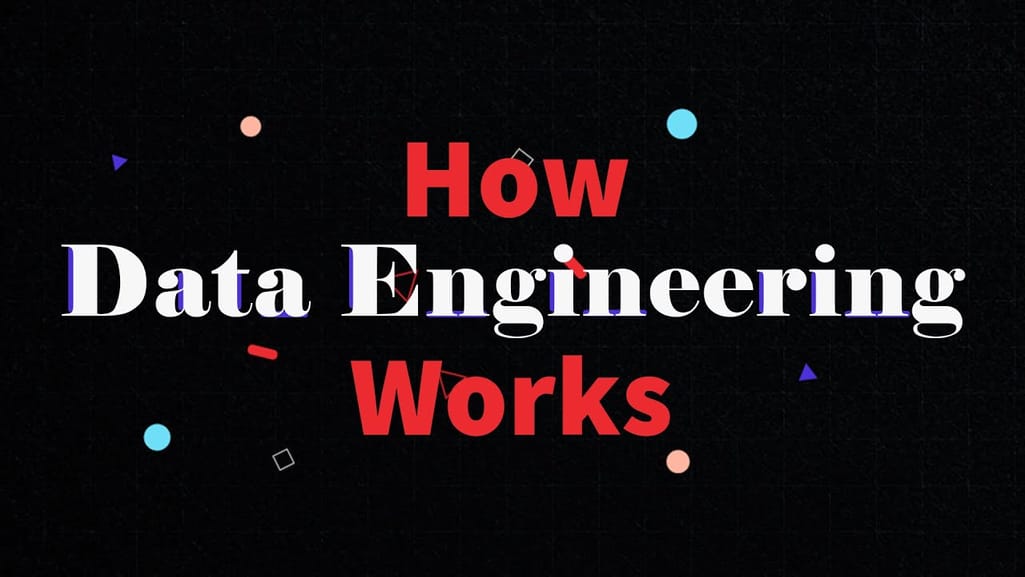
Data engineering explained
The role of a data engineer
The role of a data engineer is as versatile as the project requires. It will correlate with the overall complexity of data infrastructure. If you look at the Data Science Hierarchy of Needs, you can grasp a simple idea: The more advanced technologies like machine learning or artificial intelligence are involved, the more complex and resource-heavy data pipelines become.
The growing complexity of data engineering compared to the oil industry infrastructure. Original picture: hackernoon.com
To give you an idea of what a data infrastructure can be, and before we talk about which tools are used to handle data, let’s quickly outline three critical stages data undergoes before business users see it in the form of readable reports or dashboards.
- Extracting data. Information is all around us, but to make use of it, we have to extract it from its sources. In terms of corporate data, the source can be some database, an internal ERP/CRM system, etc. Data can come from IoT sensors scattered, say, across an aircraft or a manufacturing facility. Information may also be obtained from public sources available online.
- Transformation. Raw data will not make much sense to end users, because it’s hard to analyze it in its initial form. The transformation stage is about cleaning, structuring, and formatting data sets to make them consumable for analysis and reporting.
- Loading and storage. We need to keep extracted data somewhere. In data engineering, the concept of a data warehouse relates to the most common type of repository for data gathered for analytical purposes. But sometimes more complex data pipelines are developed that include such elements as data lakes, data lakehouses, data marts, and so on.
ETL is the most traditional architectural approach to data pipelines
The above-mentioned processes in this particular order make up Extract, Transform, Load – or ETL pipeline, the most common data pipeline type. However, sometimes the order of stages is different and the pipeline is referred to as ELT – Extract, Load, Transform. You can read more about the differences between the ETL and ELT approaches in our dedicated post. For the current discussion, it’s important that the data engineer is the specialist who designs, manages, and optimizes data infrastructure, building pipelines based on the current needs.
The responsibilities of a data engineer can correspond to the whole system at once or each of its parts individually.
General role. A data engineer found on a small team of data professionals would be responsible for every step of data flow. So starting from configuring data sources to integrating analytical tools — all these systems would be architected, built, and managed by a general-role data engineer.
Warehouse-centric. Historically, the data engineer was responsible for using SQL databases to construct data storage. This is still true today, but warehouses themselves have become much more diverse. So there may be multiple data engineers, some of them solely focusing on architecting a warehouse. The warehouse-centric data engineers may also deal with different types of storage (NoSQL, SQL), tools to work with big data (Hadoop, Kafka), and integration tools. We’ll talk about all of these aspects further on.
Pipeline-centric data engineers would take care of data integration tools that connect sources to a data warehouse. These tools can either just load information from one place to another or carry more specific tasks. For example, they may include data staging areas, where data arrives prior to transformation. Managing this layer of the ecosystem would be the focus of a pipeline-centric data engineer.
Data engineer responsibilities
Regardless of the focus, data engineers have similar responsibilities. This is mostly a technical position that combines knowledge and skills in computer science, engineering, and databases.
Architecture design. At its core, data engineering entails designing the architecture of a data platform (though in big companies with complex data processes, there might be a separate role for a data architect).
Development of data-related instruments. As a data engineer is a developer role in the first place, these specialists use programming skills to build, customize, and manage integration tools, databases, warehouses, and analytical systems.
Data pipeline maintenance/testing. During the development phase, data engineers would test the reliability and performance of each part of a system. Or they can cooperate with the testing team. They are also in charge of automating data pipelines by setting up data orchestration.
Machine learning algorithm deployment. Machine learning models are designed by data scientists. Data engineers are responsible for deploying those into production environments (but again, in some companies this is in the ML engineers’ area of competence). This entails providing the model with data stored in a warehouse or coming directly from sources, configuring data attributes, managing computing resources, setting up monitoring tools, etc.
Manage data and metadata. Information in a data repository is stored either in a structured or unstructured form. In addition, there’s metadata – or information about the data itself. Data engineers are in charge of managing all kinds of data, especially in case there’s no separate role of metadata manager.
Provide data access tools. In some cases, data engineers take on the role of BI developers and set up tools to view data, generate reports, and create visuals. We’ll talk more about the differences between these and other data-related roles in one of the next sections.
Track pipeline stability. Monitoring the overall performance and stability of the system is really important as long as the warehouse needs to be cleaned from time to time. The automated parts of a pipeline should also be monitored and modified since data/models/requirements can change.
Data engineer skills and toolset
Skills for any specialist correlate with the responsibilities they’re in charge of. The required skill set would vary for every project or organization, as there is a wide range of things data engineers could do. But generally, their activities can be sorted into three main areas: engineering, database/warehouse management, and data science.
A core skill set of a data engineer
Engineering skills
For a successful data engineering career, having a software engineering background is essential. A data engineer must be proficient in one or several programming languages. Most tools and systems for data analysis/big data are written in Java (Hadoop, Apache Hive) and Scala (Kafka, Apache Spark). Python has gained great popularity and is now the most used language for ML projects so data engineers often master it first. High-performant languages like C/C# and Golang are also popular among data engineers, especially for training and implementing ML models.
Database/warehouse knowledge
As we said, data engineers usually have to deal with different types of data storage. These include SQL and NoSQL databases, so data engineers must know how to handle them and be familiar with various database management systems like MySQL, Oracle, PostgreSQL, MongoDB, and others.
A data warehouse is the essential component of most data pipelines so knowledge of modern cloud-based warehouse technologies such as BigQuery, Snowflake, Firebolt, and Amazon Redshift is crucial for data engineers to choose the best possible warehouse architecture, set it up, and maintain it properly.
Data-related expertise
Data engineers would closely work with data scientists. A strong understanding of data modeling, algorithms, and data transformation techniques are the basics to working with data infrastructures. Data engineers will most likely be in charge of building ETL/ELT pipelines, data storage, and analytical tools. So experience with the existing ETL and BI solutions is a must.
More specific expertise is required to take part in big data projects that utilize dedicated instruments like Kafka or Hadoop. If the project is connected with ML and AI, data engineers must have experience with ML libraries and frameworks.
Summing up, data-related expertise can involve
- a strong understanding of data science concepts;
- expertise in data analysis;
- hands-on experience with ETL/ELT tools (IBM DataStage Informatica Power Center, Oracle Data Integrator, Talend Open Studio);
- BI tools knowledge (Tableau, Microsoft Power BI);
- big data technologies (Hadoop, Kafka); and
- ML frameworks and libraries knowledge (TensorFlow, Spark, PyTorch, mlpack).
Soft skills
Just like with any other role – even in tech – there are also a number of soft skills essential for best performance. For a data engineer, the most important ones are creativity and problem-solving as a big part of their daily work is looking for optimal (and often innovative) solutions to complicated issues.
Data engineers must be highly attentive to detail, be able to carry out monotonous tasks, and find ways to decompose big problems into smaller, achievable steps. Communication skills are also desirable to successfully collaborate with team members and other stakeholders.
As we already mentioned, the level of responsibility would vary depending on team size, project complexity, platform size, and the seniority level of an engineer. In some organizations, the roles related to data science and engineering may be much more granular and detailed. Let’s have a look at the key ones and try to define the differences between them.
Data specialists compared: data scientist vs data engineer vs data architect vs ETL developer vs BI developer
Data scientists are usually employed to deal with all types of data infrastructures across various organizations. Data engineers, ETL developers, and BI developers are more specific jobs that appear when a data ecosystem gains complexity. And the more complex it is, the more granular the distribution of roles becomes.
For instance, organizations in the early stages of their data initiative may have a single data scientist who takes charge of data exploration, modeling, and infrastructure. As complexity grows, you may need a team of dedicated specialists for each part of the data flow.
We’ll briefly recap on the main role distinctions here, but if you want a more detailed overview, read our article explaining the difference between data scientists and data engineers.
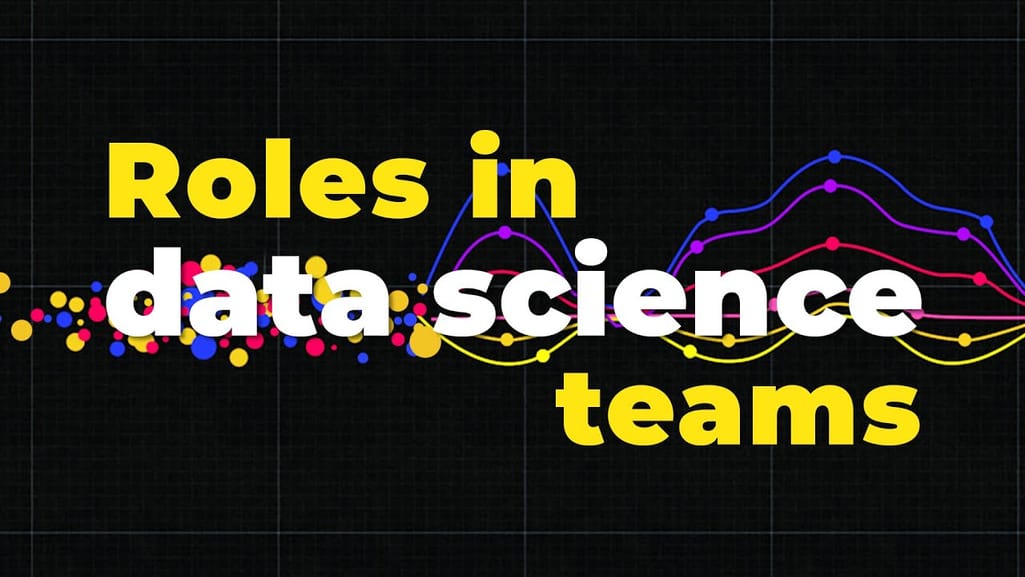
Roles in data science teams explained
Data scientists are the basis for most data-related projects. These are the specialists knowing the what, why, and how of your data questions. They would provide the whole team with an understanding of what data types to use, what data transformations must happen, and how it will be applied in the future. The input provided by data scientists lays the basis for future data infrastructure. Plainly, a data scientist would take on the following tasks:
- define required data types,
- find data sources/mine data,
- define data gathering techniques,
- set standards for data transformation/processing,
- develop machine learning models, and
- define processes for monitoring and analysis.
A data engineer is a technical person who’s in charge of architecting, building, testing, and maintaining the data infrastructure as a whole. Depending on the project, they can focus on a specific part of the system or manage overall data infrastructure. In the case of a small team, engineers and scientists are often the same people. But as a separate role, data engineers implement infrastructure for data processing, analysis, monitoring applied models, and fine-tuning algorithm calculations.
A data architect, as we mentioned earlier, is a more strategic role that usually appears in large organizations. It involves designing and overseeing the entire data infrastructure – which is then implemented and maintained by data engineers. Data architects are the ones responsible for working with business requirements and translating them into the company’s data infrastructure. They also set a data governance policy, implement DataOps strategies, and ensure data security and compliance with regulations.
An ETL developer is a specific engineering role within a data team that mainly focuses on building and managing tools for ETL stages. So the border between a data engineer and an ETL developer is kind of blurred. However, an ETL developer is a narrower specialist rarely taking architect/tech lead roles. Here’s a list of tasks that an ETL developer typically performs:
- ETL process management,
- data warehouse architecting,
- data pipeline (ETL tools) development,
- ETL testing, and
- data flow monitoring.
A business intelligence developer is a specific engineering role that exists within a business intelligence project. Business intelligence (BI) is a subcategory of data science that focuses on applying data analytics to historical data for business use. While a data engineer and ETL developer work with the inner infrastructure, a BI developer is in charge of
- defining reporting standards,
- developing reporting tools and data access tools,
- constructing interactive dashboards,
- developing data visualization tools,
- implementing OLAP cubes,
- validating data,
- testing user interface, and
- testing data querying process.
So, theoretically, the roles are clearly distinguishable. In reality however, the responsibilities can be mixed: Each organization defines the role of the specialist on its own. Everything depends on the project requirements, the goals, and the data team structure. The bigger the project and the more team members there are — the clearer the responsibility division would be. And vice versa, smaller data infastructure requires specialists who perform more general tasks.
When to hire a data engineer?
If you are a business owner or executive who realizes the value of data and builds a data-driven organization, engaging skilled experts is inevitable. There are several scenarios when you might need a data engineer.
Scaling your data science team. Here’s a general recommendation: When your team of data specialists reaches the point where you lack people to develop and/or maintain a technical infrastructure, a data engineer might be a good choice in terms of a general specialist.
Running big data projects. Currently, data engineering shifts towards projects that aim at processing big data, managing data lakes, and building expansive data integration pipelines. In this case, a dedicated team of data engineers with allocated roles by infrastructure components is optimal.
Requiring custom data flows. Even for medium-sized corporate platforms, there may be the need for custom data engineering. ETL is just one of the main principles applied mostly to automated BI platforms. As we mentioned, in practice, a company might leverage different types of storage and processes for multiple data formats. This involves a large technological infrastructure that can be built and managed only by a versatile data specialist. A data engineer in this case is much more suitable than any other role in the data domain.
How to become a data engineer: education, background, data bootcamps, and certifications
We also have some recommendations for those of our readers who are considering building a career in data engineering.
Education. Most employers are looking for specialists with at least a bachelor’s degree in some technical field (e.g., computer science or information technology) that develops such essential skills as coding, problem-solving, and analytical thinking. Getting a master’s degree will definitely boost your professional development and unlock higher positions.
Background. As we said earlier, this role requires heavy technical knowledge so a solid background in IT (computer science, engineering, applied mathematics, etc.) can be a great starting point for your data engineering career. Experience with multiple programming languages, especially Python and Java, is usually essential. Knowledge of database systems (SQL and NoSQL), data warehouses and data lakes, distributed computing frameworks, ETL/ELT technologies, and cloud data platforms would be a huge plus.
Data science bootcamps. If you have an unrelated degree and want to get the skills needed to become a data engineer quickly, consider enrolling in a data science bootcamp. They typically take a few months, have a variety of learning formats (online/offline, full-time/part-time, etc.), and are way cheaper than a college degree. However, be prepared for intensive, immersive learning packed with classes on statistics, machine learning, programming languages, data tools, and so on.
Some data science bootcamps you can check out are DataCamp, The Data Incubator, MIT xPRO, and DataScientest.
Certification. Despite being one of the most in-demand positions in the tech industry, a data engineer is still considered a new role so there aren’t too many focused certifications yet. Here are some of the existing industry-recognized ones.
- Google Cloud Professional Data Engineer (there’s also a number of related courses you can check out)
- Cloudera Certified Professional Data Engineer
- IBM Certified Data Engineer
- Associate Big Data Engineer by DASCA
You can also be accredited in a related sphere (like data analytics), get a cloud certification (AWS/GCP/Azure), or master data platforms (Databricks/Snowflake/BigQuery). Having any of those in your resume will help you launch a successful and lucrative career in data engineering.