Projections show that the data governance market will expand from $1.81 billion in 2020 to $5.28 billion by 2026. More solutions and services are becoming available, so if you haven’t established a data governance framework yet, now is the perfect time.
In this article, we will explore what data governance is, the key components of a data governance framework, and best practices for implementing a successful data governance strategy. Whether you're just getting started with data governance or looking to refine your existing program, this article will provide you with valuable insights and practical tips to help you manage your data more effectively.
What is data governance?
Data governance is a broad term that refers to a critical set of organizational policies, processes, roles, standards, and metrics for ensuring the accuracy, consistency, security, and availability of data as well as its effective use.The Data Governance Institute defines it as “a system of decision rights and accountabilities for information-related processes, executed according to agreed-upon models which describe who can take what actions with what information, and when, under what circumstances, using what methods.”
The Institute also highlights the necessity to be cautious when using the term governance as it can have different meanings based on context.
Key areas where data governance brings value
Data governance fields of action vary from organization to organization, and there is no fixed set of tasks that applies to all. However, certain areas are commonly addressed in data governance, such as those listed in the following examples.Data quality involves storing data in its correct and consistent form. Here’s a deep dive into data quality management and tools.
Data availability is responsible for making data accessible to appropriate personnel within the system.
Data usability ensures that data is available in a structured format that is compatible with traditional business tools and software.
Data integrity is about maintaining the quality of data as it is stored, converted, transmitted, and displayed. Learn more about data integrity in our dedicated article.
Data security is the set of processes that prioritize certain data based on its sensitivity and implement measures to protect it across all online and offline platforms.
Data modeling involves creating a conceptual representation of data objects and their relationships to each other, as well as the rules governing those relationships.
To design an effective data governance program, it's crucial to choose an operational model that fits your business size and structure.
Data governance models
There are three basic data governance models — centralized, decentralized, and hybrid. The specific data governance model that an organization adopts depends on various factors, such as its size, complexity, industry, and regulatory environment.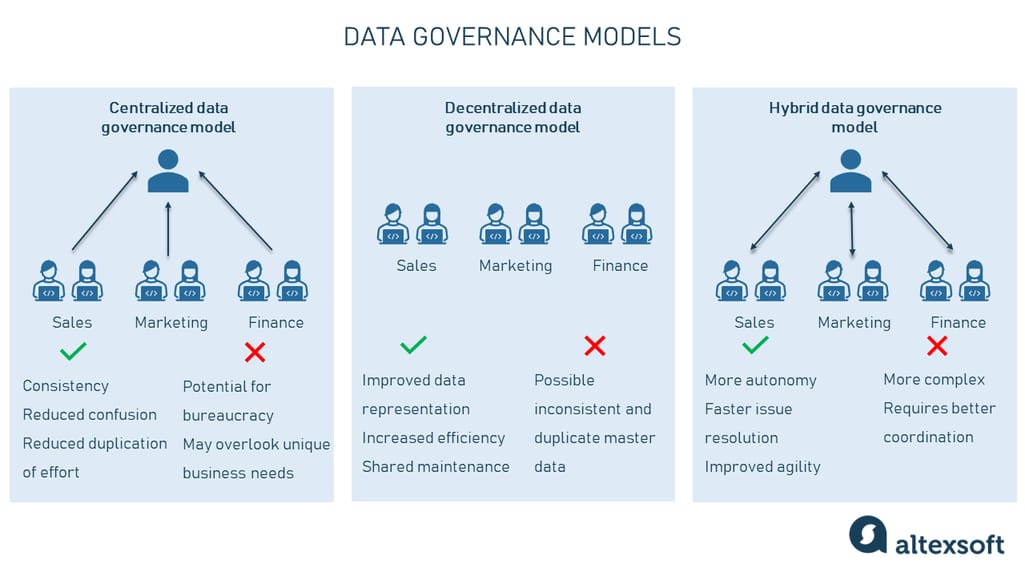
Data governance models with their pros and cons.
Centralized governance model. A central data governance team or lead manages the organization's data assets and establishes policies, processes, and standards for data use and management. The beneficial part of centralized governance is that it allows for consistent standards and definitions to be applied to data across the organization, reducing confusion and duplication of effort. However, centralized models may fuel bureaucracy and overlook the unique needs of different areas of the business.Decentralized governance model. Different business units within the organization have their own data governance teams and are responsible for managing their data assets. Benefits include improved representation, better data, increased efficiency, and shared maintenance. The latter is a set of consistent and reliable data entities that are shared across an organization and used to support business processes and decision-making. However, decentralized models may result in inconsistent and duplicate master data.
Hybrid (federated) governance model. There’s a centralized structure that provides a framework that is then used by autonomous departments that own their data and metadata. Benefits include more autonomy, faster issue resolution, and improved agility. However, a hybrid model requires a centralized body to have deep expertise in each business area and coordination to ensure data consistency.
Ideally, despite the model chosen, data governance covers all strategic, tactical, and operational aspects of data management, which brings us to the necessity to distinguish these and other terms.
Data governance vs data management vs data stewardship vs master data management
The term data governance is often mistaken for concepts like data management, data stewardship, and master data management. It's crucial to differentiate between these terms as each plays a distinct role in ensuring the proper handling, use, and protection of data.Data management is the overall process of collecting, storing, organizing, maintaining, and using data. Essentially, the fundamental principle underlying this process is to recognize data as a valuable resource, given its significant role in driving business success. Data management is a technical implementation of data governance and involves the practical aspects of working with data, such as data storage, retrieval, and analysis.
Data governance, on the other hand, focuses on the strategic and organizational aspects of managing data, such as establishing policies and procedures for data use and ensuring compliance with regulatory requirements. A component of data management, data governance is a higher-level strategic concept that also encompasses elements such as data quality, data privacy, and data security.
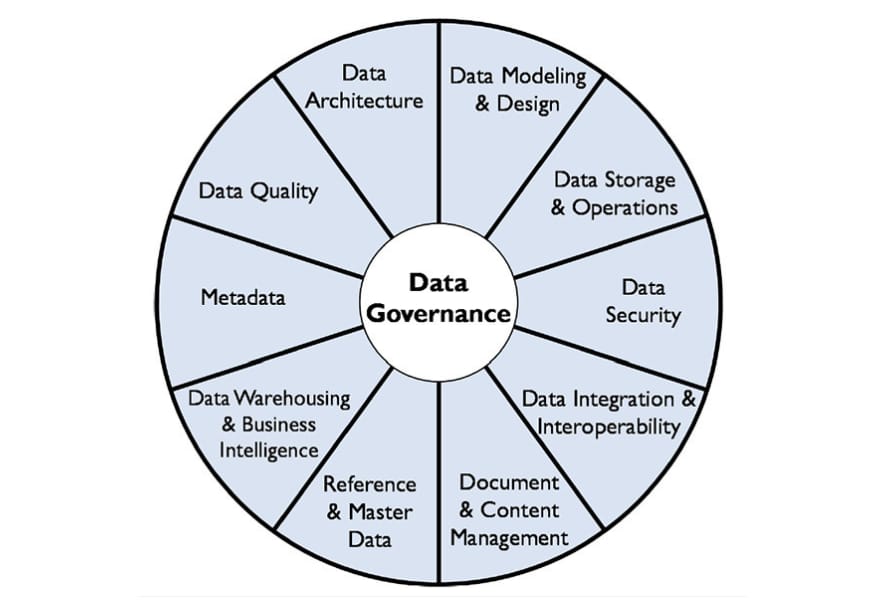
The DAMA DMBOK wheel. Source: DAMA International
DAMA International has published the Data Management Body of Knowledge (DMBOK) which is a model for data management where data governance resides in the hub of the wheel.Data stewardship is a subset of data governance that involves management and oversight of the data assets of an organization. Data stewards are responsible for ensuring that data is accurate, complete, and consistent with organizational policies and standards.
Master data management (MDM) and data governance are related but distinct practices in managing data. MDM focuses on improving the quality of specific business-critical types of data, such as customer or supplier data, and ensuring that they are consistent across the organization. On the other hand, data governance provides a broader framework for managing all data, including defining data models, retention policies, and roles and responsibilities. While MDM is a subset of data governance, proper governance is crucial for successful MDM implementation.
In essence, data management and data governance are different but complementary concepts that work together to guarantee that data is effectively and efficiently managed within an organization. Data governance provides the overall framework for managing data with data stewardship being a part of this, while data management focuses on the practical implementation of data-related tasks.
Why opt for data governance?
Effective data governance is crucial for ensuring the reliability and accuracy of data within your organization. Without it, data may become invalid or difficult to access, hindering employees' productivity and leading to non-value-added activities.In case you haven’t implemented data governance yet, below are a few arguments in favor of starting to think this way.
A common understanding of data. Data governance creates a consistent view of data, establishing a common terminology while allowing individual business units to retain appropriate flexibility.
Improved quality of data. Data governance creates a plan that ensures data accuracy, completeness, and consistency.
A survey by McKinsey revealed that poor data quality and availability resulted in up to 30 percent of total enterprise time being spent on non-value-added tasks, with variations observed across different departments and roles.
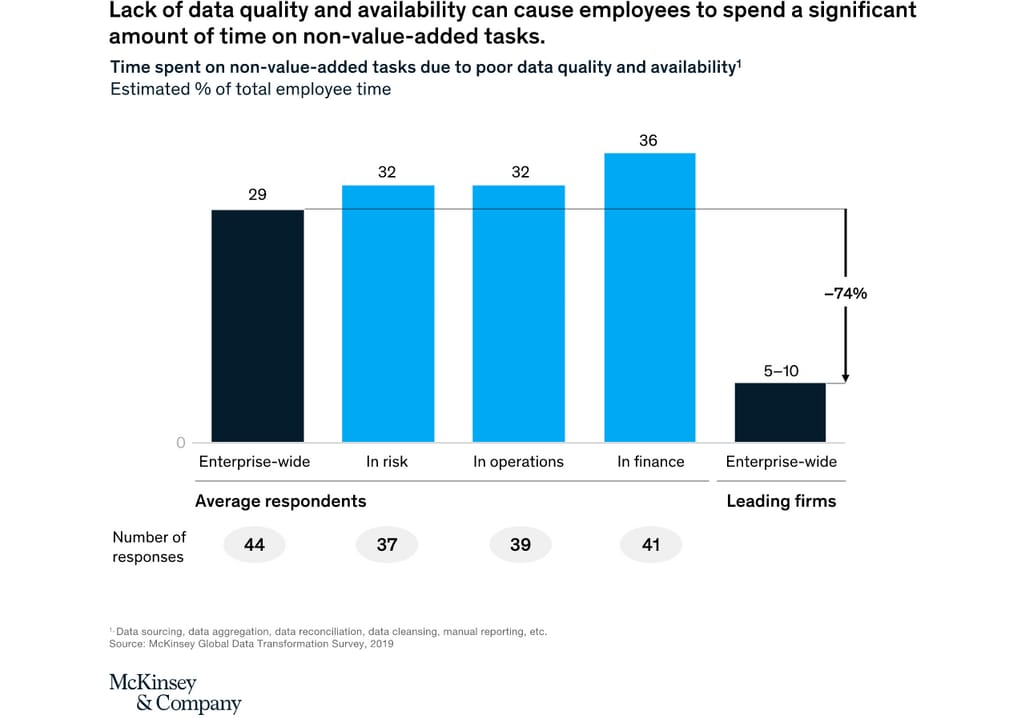
Time spent by enterprise employees on non-value-added tasks due to bad quality and unavailability. Estimated percent of total employee time. Source: McKinsey&Company
For example, a data science team may spend 70 to 80 percent of their time preparing data for machine learning projects, with the prevailing part of this time being spent on data cleansing alone.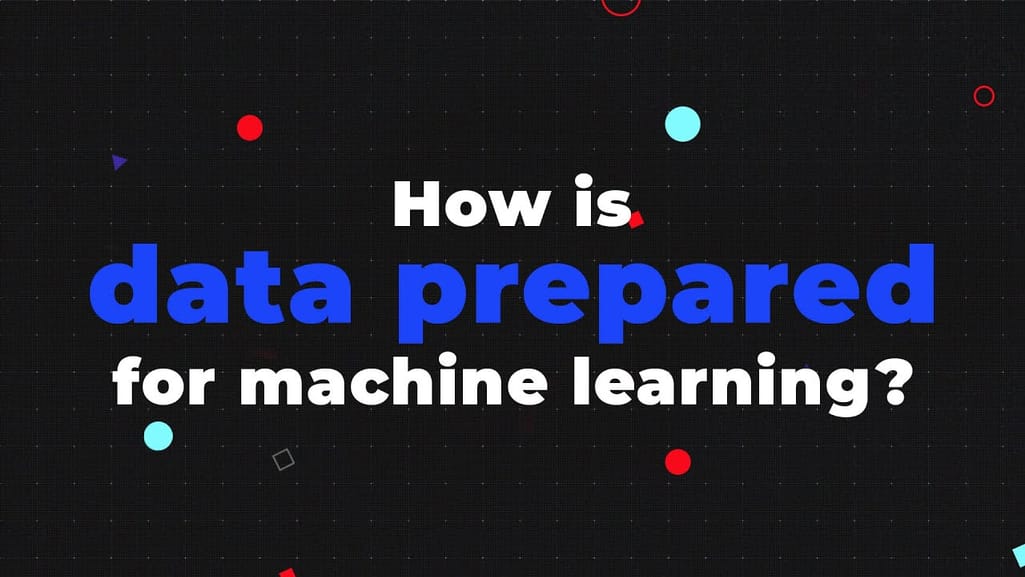
Learn how data is prepared for machine learning in our dedicated video.
Data quality management can help make employees 25 percent more productive, a significant improvement. Thus, strategically applying data quality management processes is crucial for any organization.
Data discoverability. Data governance provides an advanced ability to understand the location of all data related to key entities, making data assets usable and easier to connect with business outcomes.
A 360-degree view of each customer and other business entities. Data governance establishes a framework for agreeing on "a single version of the truth" for critical business entities and creating an appropriate level of consistency across entities and business activities. With a complete customer view, businesses can target them more efficiently and capitalize on timely upselling and cross-selling opportunities.
Compliance. Data governance provides a platform for meeting government regulations like GDPR or HIPAA. For instance, if an organization's goal is to maintain the privacy of healthcare-related data, it must ensure that patient data is handled securely as it moves through the organization. Data governance puts in place all the needed requirements that keep a record of who made changes to data and when to ensure compliance with HIPAA.
Improved data management. Data governance brings the human dimension into a highly automated, data-driven world. It establishes best practices in data management, making certain that concerns and needs beyond traditional data and technology areas — including fields such as legal, security, and compliance — are addressed consistently.
Access and documentation. One of the most fundamental aspects of data governance is accessing and documenting data. When initiating new data projects, it is crucial to identify the types of data available — structured, unstructured, or semi-structured. With a proper understanding of data sources, their content, processing methods, and usage, you can achieve clarity and transparency and facilitate the efficient implementation of data-based products.
All the above-mentioned prove that organizations can enhance data management and support better business outcomes with an effective data governance strategy in place.
Components of a data governance framework
It's not possible to simply purchase a ready-made data governance program, which can be both a good and a bad thing. Organizations must tailor their program to their needs, budget, and capabilities. Regardless of how much of a data governance program is implemented, it's essential to have a data governance framework — a basic set of guiding concepts and a structure to apply them.
While there are various framework options to choose from, they all have common components that address people, processes, and technology. We’ve rounded up the key elements of a data governance framework.
Key components of a data governance framework.
Strategy
Data governance strategy is a key element of the framework, which involves creating a vision and mission for managing data effectively.
The vision provides a clear understanding of what the organization aims to achieve through its data governance efforts. This could include objectives such as ensuring data accuracy and consistency, improving data quality, increasing data security, and ensuring regulatory compliance.
The mission defines the specific actions and initiatives required to achieve the vision, e.g., implementing data governance policies and procedures, establishing data quality standards, identifying and managing data risks, and defining data ownership and accountability.
The strategy should also align with the overall business objectives such as improving customer satisfaction, increasing revenue, reducing costs, or achieving regulatory compliance.
Roles and responsibilities
The core of a data governance program is composed of people: data governance specialists and other key business and IT personnel who establish and maintain workflows to meet the organization's data governance requirements.
The roles in data governance can vary from company to company, but there are several stakeholders and units that are often involved in data governance activities.
The Data Governance Committee is a higher-level, cross-functional unit that consists of experts from different business areas. Its goal is to define and control all data governance initiatives. The maximum effectiveness of the Data Governance Committee is achieved when subject matter experts (e.g., data engineers, data security managers) are combined with system managers (e.g., solution architects, data analytics managers) and business units (e.g. sales specialists).
The Data Steward is the key figure responsible for overseeing the data governance initiatives and intervening or informing the involved parties about any anomalies. The data steward is often referred to as the "guardian" of the data.
The Chief Data Officer (CDO) is often considered the initiator and leader in the field of data governance. The CDO is responsible for defining and implementing data policies, standards, and procedures to ensure that data is managed in a consistent, accurate, secure, and compliant manner. The CDO also plays a key role in promoting data literacy within the organization and ensuring that data is used effectively to achieve business objectives. Sometimes the same responsibilities go to a Chief Digital Officer.
The Solution Architect is responsible for designing and implementing technology solutions that meet the business requirements of an organization, and data governance can be one of the aspects of this work. A solution architect can work closely with stakeholders to define data governance policies and procedures, design data architectures, and implement data management processes.
A Data Architect typically focuses on creating and building data infrastructures inside an organization. So a data architect can contribute to the design and implementation of data governance policies and processes so that they are aligned with the organization's data architecture. However, it is important for organizations to have a dedicated data governance team or individual who can drive the implementation of the data governance program, as it requires a broad range of skills and expertise beyond just data architecture.
Policies, processes, and standards
At the heart of every data governance program are the policies, processes, and standards that guide responsibilities and support uniformity across the organization. Each of these must be designed, developed, and deployed.
Data governance policies are a high-level set of statements that influence or direct data habits at the business level, such as outbound data sharing and regulatory compliance.
Data processes refer to the workflows and procedures put in place to manage and maintain data quality, accuracy, and consistency across an organization. They often include data quality surveillance, data issue tracking and resolution, automated data quality testing, data lineage tracking, evaluation, and other operations.
Data rules and standards ensure that data policies are followed. Data rules control or restrict activity to enable compliance with data standards that cover areas such as master and reference data, metadata management, data definitions and domain development, classification, accessibility, and more.
Metrics and measurement
To determine the effectiveness of a data governance program, it's important to establish business metrics and Key Performance Indicators (KPIs) that can be tracked and assessed consistently over time. These metrics should be quantifiable and relevant to the organization's business objectives.
It's also essential to conduct a baseline measurement before implementing a data governance program to track progress and measure success over time. Without a baseline measurement, it's difficult to determine whether the program is achieving its goals or not.
Technology
In order to maximize automation, data governance programs will need to incorporate a range of technologies. Smaller companies may rely on their existing tools. However, larger data governance projects usually require specific, enterprise-level software. We’ll explore the available instruments in a separate section.
Communication and collaboration
Effective communication is crucial in implementing data governance programs, which often involve changing and evolving requirements. It can take various forms, such as in-person meetings, emails, newsletters, and workshops. Change management, in particular, requires careful attention to ensure that all team members understand how the data governance program's changes will impact them and their responsibilities.
Data governance involves a diverse group of stakeholders who must collaborate to effectively manage data. Collaboration as well as a positive culture that rewards teamwork is essential. Clear channels of communication between teams, such as regular meetings, are necessary. Online collaboration platforms are also increasingly being used.
Data governance tools
Data governance tools come in a variety of forms with different capabilities depending on the specific needs of an organization. However, ordinarily, the functionality covers the following aspects.
Data cataloging helps in organizing and categorizing data assets across the organization, making it easier to find, access, and understand data.
Data quality management allows for monitoring and maintaining data across the organization, identifying issues, and implementing measures to improve data quality.
Data lineage provides a complete view of how data moves across the organization, from its creation to end usage, making it easier to identify and troubleshoot issues.
Access control defines who can use the data within the organization, ensuring compliance with regulatory requirements and preventing unauthorized access.
Workflow management helps in automating data governance processes, making it easier to track and handle data-related tasks, approvals, and activities.
The data privacy and security feature ensures that sensitive data is protected through access controls, encryption, and other security measures.
The reporting and analytics feature provides visibility into data governance metrics, enabling organizations to track performance, identify areas for improvement, and measure the impact of data governance initiatives.
There should also be a feature allowing collaboration between different teams and stakeholders involved in data governance initiatives.
Here are some tools on the market you can consider when deciding on implementing a data governance strategy.
Informatica Cloud Data Governance and Catalog: Suitable tool for multi-cloud businesses
Informatica Cloud Data Governance and Catalog is a cloud-based data governance tool for discovering, managing, and analyzing data assets across various cloud and on-premises environments. It helps organizations define and enforce data policies, track data lineage, and improve data quality and compliance.
Cloud Data Governance and Catalog dashboard from the demo. Source: Informatica
The tool allows users to gain insight into their data assets, ensuring they can confidently use them for data projects. It provides easy access to data lineage, enabling you to view the source of data residing in a data asset. Additionally, the tool provides different features to check the quality of a data asset as well as the policies and terms of use associated with it. For example, you as a user can pick a certain data item, see where it resides in the hierarchy, and check its relationships. The tool seamlessly integrates with other Informatica products, such as data integration and master data management technology.
Best for: Companies that operate in highly regulated industries, such as healthcare and financial services, and those that need to manage large volumes of data across various cloud and on-premises environments.
Egnyte: Easy-to-use cloud-based data governance tool for small to midsize businesses
Egnyte is a cloud-based data governance tool with features such as secure file sharing, granular access controls, and compliance with regulatory requirements.
Data lifecycle management. Source: Egnyte
Egnyte offers a variety of data governance functionality, including data classification and tagging, automated data retention policies, and audit trails to track data usage. Additionally, the tool provides real-time analytics to identify and address potential data security risks. It can also help organizations ensure compliance with regulations such as HIPAA and GDPR while enabling efficient collaboration among teams.
Best for: Small to midsize businesses (SMBs) and enterprise companies that require a cloud-based solution for managing and protecting their data. It is also suitable for companies with remote and distributed workforces that need secure collaboration tools.
SAP Master Data Governance: A helper tool for large enterprises
SAP Master Data Governance helps organizations manage their master data assets in a consistent, accurate, and reliable manner. It enables companies to establish policies, procedures, and rules for managing data while providing a framework for data quality monitoring and control.
SAP MDG dashboard from the demo. Source: SAP
SAP MDG allows for data governance to be implemented at scale. It enables collaboration between business and IT teams, ensuring that data is managed according to established policies and procedures, and that data quality is maintained throughout its lifecycle.
Some of the key features of SAP Master Data Governance include data modeling, data quality management, data change management, and data governance workflows. It integrates with other SAP solutions, such as SAP S/4HANA and SAP Business Suite, to provide a unified platform for managing enterprise data.
Best for: Companies with complex data environments, multiple business units, and regulatory compliance requirements. It is particularly useful for organizations in industries such as manufacturing, retail, and healthcare that rely heavily on accurate and consistent master data to drive their operations.
Choosing the right data governance toolset or developing a custom software product is half the battle, but it's not enough to achieve a successful data governance program. To make the most of your investment, you also must follow best practices for implementing a data governance strategy.
Best practices for implementing a data governance strategy
Here are the best practices for implementing a data governance strategy.
Start small. To avoid complications and team demotivation, it is recommended to begin your data governance program with a small-scale project instead of a complex or long-term one. Starting with a more manageable task, such as analyzing data for a single team, will help you identify the state of the data, including its collection, storage, and usage. From there, you can evaluate the project's success and determine how much budget to allocate to the initiative. This approach allows you to test the waters and gain insights before expanding your data governance program to larger and more complex projects.
Assemble a dedicated team. Establish a team of stakeholders from various departments to develop and manage the data governance program, including business leaders, IT staff, data owners, and end-users. This will ensure that the program is tailored to the organization's specific needs and objectives.
Define and communicate data governance goals. Clearly define and communicate the goals of the data governance program to stakeholders to ensure everyone is aligned and working towards a common objective.
Foster transparency with external stakeholders. It's important to be transparent with external stakeholders regarding how data is collected, stored, and used to maintain trust and avoid any negative consequences.
Integrate data governance across all departments. Embed data governance into every department to ensure that everyone understands their roles and responsibilities in managing data and to facilitate cooperation across departments.
Establish risk management milestones. Identify and establish risk management milestones to mitigate potential risks, such as data breaches or security vulnerabilities.
Conduct assessments after project completion. After completing a data governance project, assess effectiveness to identify areas for improvement and implement changes to ensure continued progress.
Continuously refine your data governance framework. Data governance is an ongoing process that requires continuous refinement to keep pace with technological advances, changes in data management practices, and evolving regulatory requirements. Continuously refine your data governance framework to ensure that it remains effective and relevant.
By following these best practices, you can develop and implement an effective data governance program that supports your objectives and ensures that data is managed securely and effectively.