Here's a great story about how easy it is to manipulate consumer demand online -- if you're skilled at crafting fraudulent reviews. In 2017, British online journalist Oobah Butler, who made money by writing fake restaurant reviews on Tripadvisor, experimented out of professional curiosity: He created a profile for a phony restaurant called "The Shed at Dulwich" and began promoting it.
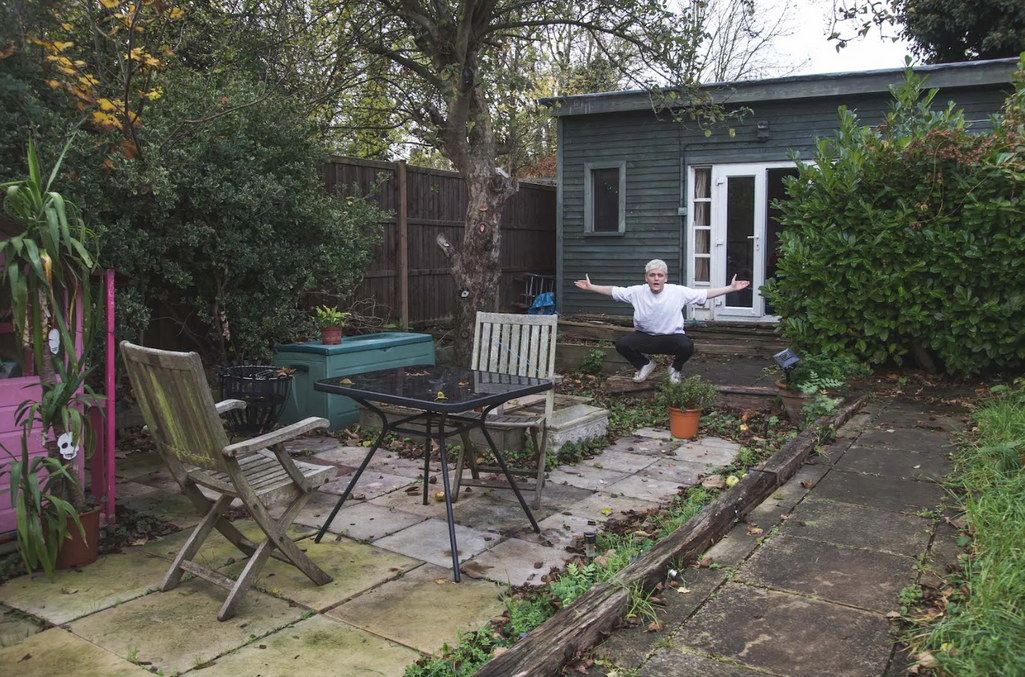
Oobah Butler setting his fake restaurant at the shed at Dulwich, London. Source: Vice
In just six months, the magic of fabricated feedback catapulted this nonexistent diner to the top of the London restaurant list. Butler became so overwhelmed with calls from people begging to book a table that he eventually set up a couple of tables in his backyard, bought ready meals from a supermarket, and hosted a dinner for a few eager customers. Amazingly, people were satisfied and planned to return. “The odor of the internet is so strong nowadays that people can no longer use their senses properly,” noted the prankster, astonished by the impact he had originated.
Later, the journalist contacted Tripadvisor to tell them about the incident. A company representative replied that "the only people who create fake restaurant listings are journalists in misguided attempts to test us" and that "most fraudsters are only interested in trying to manipulate the rankings of real businesses." Which was fair enough, but it didn't solve the problem.
Fake reviews don't just exist; they can elevate worthless businesses to the top of the food chain, even if only for a moment of glory, and cause irreparable damage to honest market players. And although big companies invest a lot of money and time in fake review detection, this process is more like a cat-and-mouse game.
This article will explain why fake reviews are dangerous for your business and how to fight them using ready-made tools or a machine-learning model tailored to your business needs.
How fake reviews became a problem
People naturally tend to trust word of mouth more than billboards, and today, user-generated reviews are like word-of-mouth on steroids. An average user reads between one and six customer reviews before parting with money.
Online feedback has become a significant factor in the digital economy: Approximately 80 percent of Tripadvisor users are more inclined to book a hotel with a higher rating when selecting between two identical properties. Additionally, over half (52 percent) say they would never book a hotel without reviews. An extra star on a restaurant's Yelp rating can boost revenue by 5-9 percent. Unsurprisingly, as soon as online feedback became a thing, individuals and companies started trying to manipulate it.
There are several ways to game the system.
- Posting phony positive feedback to boost a product rating, can be done by somebody connected to the business (an owner or an employee) or by an outsider hired for this task.
- Writing false negative comments about competitors, also called vandalizing.
- Spamming with irrelevant content not related to the product being reviewed.
Fake reviews aren’t always written for money; sometimes, they stem from personal bias, sociopathy, or just a bad mood. The main characteristic of fraudulent reviews is that their authors have never actually bought or used the goods or services they're giving opinions about.
Fake reviews should be distinguished from incentivized reviews written at the business's request by users with real product experience. Customers might be offered discounts or reward points for their time, but the reviews are assumed to be unbiased. However, many platforms like Google, Meta, Yelp, and Tripadvisor prohibit this practice, believing any incentive influences the review's content, usually favoring the brand. This means such reviews can't be considered authentic or trustworthy.
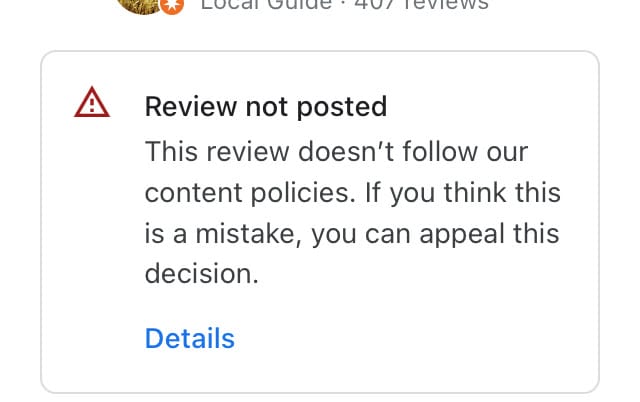
Google’s Review System Algorithm filters reviews it considers biased. Source: Google Help
According to the Tripadvisor Review Transparency Report, about 4.4 percent of all reviews submitted to the platform in 2022 were fake, up from 3.6 percent in 2020 and 2.4 percent in 2018. India and Russia top the list of countries producing fake reviews. Note that these numbers only reflect the proportion of fakes the platform identified and removed. The phony restaurant story shows that algorithms to detect and filter out such reviews are not foolproof.
The state of affairs is no better on eCommerce platforms: 10-15 percent of published reviews are fake.
Over the past two years, the situation has been exacerbated by ChatGPT's constant readiness to scribble dozens of fake reviews per second to help content farms.
Why are fake reviews dangerous to your business?
Any deceptive reviews, whether positive, negative, or simply spam, distort consumer experience and pose significant dangers for both businesses and consumers.
Positive fake reviews create a false impression of a business's quality. Let's say a small hotel has overblown its Google Maps, Tripadvisor, and Facebook pages with rave reviews. Guests expecting top-tier service find the reality falling short, resulting in customer disappointment and damage to reputation.
If you have encountered similar problems, this article about online reputation management for hotels might be useful for you.
Fabricated negative reviews can lead to an immediate drop in sales and long-term brand degradation. For instance, California-based Super Mario Plumbing reported a 25 percent drop in business due to a fake review posted by a competitor.
Spam reviews clutter review platforms with irrelevant or misleading information, making it difficult for potential customers to find authentic feedback and decide to make a purchase.
Finally, a company manipulating reviews risks facing both commercial and legal sanctions. Major platforms that detect fraudulent reviews often mark the company’s profile as unreliable and may suspend or remove it.
Regulatory bodies like the Federal Trade Commission can penalize companies or individuals for creating and spreading false reviews. In 2022, online fashion retailer Fashion Nova paid a $4.2 million fine to the FTC for using a third-party tool that automatically posted positive comments on their website while blocking ratings below three stars. By the way, the Commission is working on a new trade rule that holds businesses liable whether they paid for fabricated feedback themselves or failed to notice it on their website or platform in time. Fines can be as high as $50,000 each time a consumer sees a fake review.
That all sounds pretty unpleasant. Suppose you're an entrepreneur or executive at a company that depends on online reviews (i.e., restaurant, hotel, OTA, eCommerce store, or service provider). In that case, you'd better be concerned about protecting your brainchild by identifying and defusing fake reviews.
Fake review detection can be done manually or automatically, but the former is time-consuming and less accurate. Studies show that humans can only spot fake reviews about 57 percent of the time, whereas artificial intelligence may approximate 90 percent of predictive accuracy. Therefore, not only giants like Amazon, Google, Tripadvisor, and Trustpilot but also small and medium-sized businesses rely on machine learning models to identify fraudulent reviews.
Read about credit card fraud detection and how machine learning can protect businesses, or watch the video on fighting financial scams.
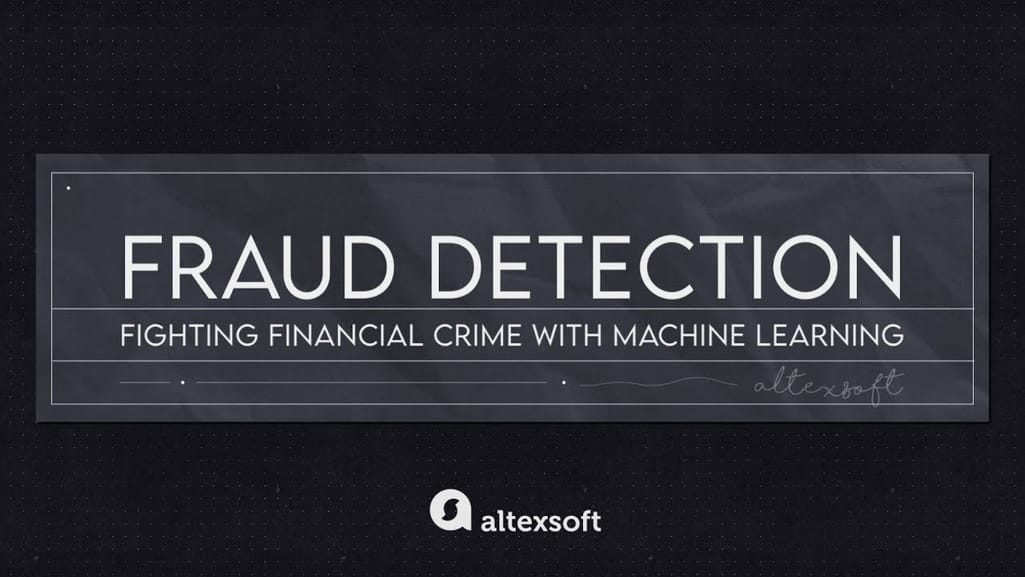
Fake review datasets
Let's say your company has decided to build a custom ML-driven detector for false reviews. Then the first step is to acquire data to teach your model how to spot fakes.
Custom data collection and preparation
The largest sources of data you’re looking for are review aggregators, such as Yelp, Tripadvisor, Google, and others. Technically, you can collect comments they accumulated manually or automatically — with web scraping tools (e.g., Google Maps Reviews Scraper) or via APIs if a platform offers an API access to its data. In any event, you must contact a provider first to get written permission to gather web data or set up API integration.
Read our article on data collection for machine learning to dive deeper into the topic.
But the key question is: How do you distinguish fake reviews from real ones? Your future model must learn to tell them apart. For that, you need a dataset with reviews labeled as real or fake.
One approach is to have your data science team analyze the public reviews and separate the fake ones from the real ones.
Pay attention to the following signs of fake reviews.
The date. A surge of reviews immediately after a product release can indicate fakes, and reviews predating the release are suspicious.
Lack of verified purchase. Fake reviews frequently lack a "Verified Purchase" label, indicating the reviewer may not have actually bought the product.
High frequency of reviews. Reviewers with an unusually high number of reviews in a short period may be writing fakes.
Other reviews of the same user. Repeated phrases and all five-star ratings are suspicious.
Characteristics of the profile. Fraudulent profiles often have generic names, like John Smith, random strings (#3545!), or no profile pictures.
Overuse of certain words. Fake reviews overuse me, I, and verbs to seem credible. Organic reviews often contain more nouns.
Scene-setting. Fake reviews often describe personal scenarios (like birthday parties, gifts for an anniversary, and so on). Genuine reviews focus more on specific product details.
Spelling and grammar. Poor language skills often indicate a review from a low-quality content farm.
Black and white reasoning. Extreme love or hate can signal fakery. Genuine reviews are more nuanced.
Praise a competitor. Negative reviews that praise a competitor might be fake, promoting another business.
But since fake reviews can be really hard to sort out, ML projects in this field often rely on datasets augmented with artificially generated or synthetic data. For example, a crowdsourcing marketplace, Amazon Merchant Turk, was engaged to write deceptive opinions to supplement Tripadvisor’s dataset of Chicago hotel reviews, which was used in many research projects on detecting fake reviews.
Once you have a sufficient number of verified true and fake reviews, you should clean the data. For texts, this step includes removing irrelevant or meaningless elements (special characters, whitespaces, and stopwords), converting all characters to lowercase, and reducing words to their stems.
Read our article on how to prepare data for machine learning to dive deeper into the subject or watch a video explainer.
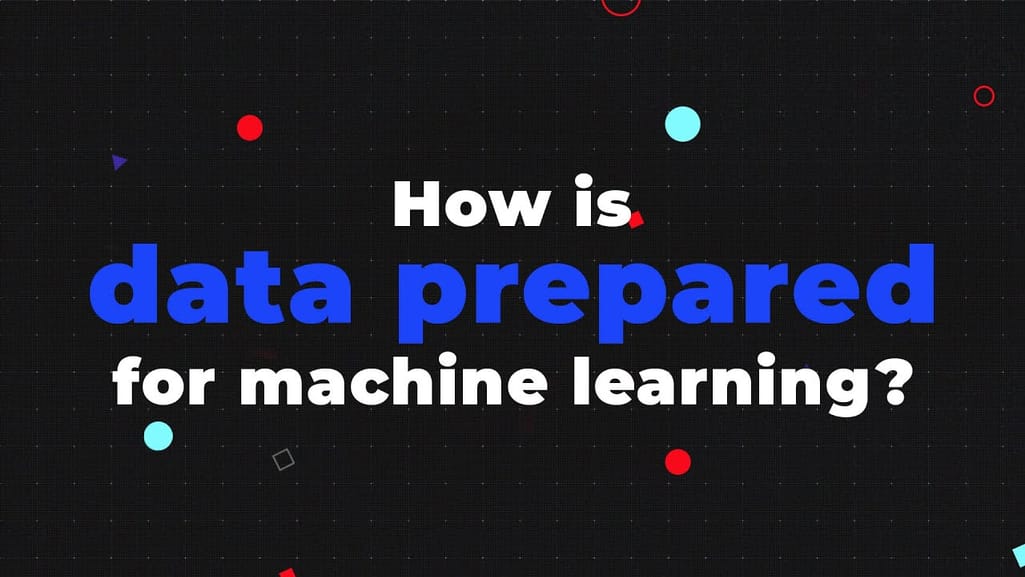
How is data prepared for machine learning?
Data analysts may suggest supplementing the dataset with other features useful for distinguishing a genuine review from a fraudulent one. For instance, these could be star ratings, helpfulness scores, timing, frequency, number of reviews from the same user, IP addresses, GPS data, social networks (relations between review authors in social media), product images, sentiment scores (the level of emotionality based on word usage like happy, sad, excellent, terrible, love, hate, etc.), and more.
Third-party datasets
Instead of collecting or generating data yourself, you can get an off-the-shelf dataset.
Yelp Open Dataset includes businesses, reviews, and user data for academic use, but for commercial purposes, you have to contact the platform and require permission.
Kaggle has various datasets of reviews (for example, Trip Advisor Hotel Reviews) with clear licensing terms allowing commercial use.
SentiOne specializes in collecting and analyzing online conversations, including reviews, social media posts, and other user-generated content. It also provides data for commercial use.
Some other public datasets are available through open data initiatives like UC Irvine Machine Learning Repository and sometimes permit commercial use, depending on their licensing terms.
Finally, many researchers specializing in fake review detection create datasets for academic purposes, including those collected on Amazon, Tripadvisor, IMDB (movie reviews), etc, which may be suitable for your purposes — you just need to negotiate the terms of use with the creators.
Machine learning models for detecting fake reviews
When you have a dataset, the next step is to build a machine learning model. You'll train it using 80 percent of the data and set aside the remaining 20 percent to test it. But before anything else, you must decide on an algorithm that will be the foundation of your predictor. Typically, data science teams experiment with several methods and their combinations to choose the option that produces the most accurate results.
Below, we’ll look into algorithms most frequently used in academic studies related to this topic. Methods applied in real-life settings are not typically disclosed for safety reasons.
Support Vector Machines
These robust classifiers divide data into two categories by finding the hyperplane that best separates different classes in a high-dimensional space. An SVM-based fake review detection system applied on a publicly available dataset of hotel reviews achieves an accuracy of 95.6 percent.
Pros. It performs well with many features expected in text data, is suitable for complex text features, and is resistant to overfitting — an undesirable change in the algorithm's performance after introducing new data.
Cons. The method can be slow to train, especially with large datasets.
Naive Bayes Classifiers
This family of algorithms has its roots in a formula created by 18th-century mathematician Thomas Bayes to find the probability of events knowing prior conditions. All classifiers from the group share the simplified or naive assumption that features in the input data are independent of each other.
For example, the attribute "non-verified/verified user" has nothing to do with and doesn’t impact the likelihood of all other variables (time of posting, frequency, IP address, etc.) in a fake or true class. All features in this scenario have the same importance (which can be untrue in real-life settings.)
The bag-of-words approach (treating a text as a collection of individual words without considering their order) makes this algorithm highly effective for text classification like spam detection. The Naive Bayes model trained on the Amazon Customer Reviews dataset showed an accuracy of up to 99 percent in sentiment analysis.
Pros. It is high-speed and easy to implement.
Cons. Simplicity can be a drawback for capturing complex patterns in data.
Random Forests
This method combines multiple decision trees. Individual decision trees are prone to overfitting because they capture all noise and random fluctuations in the training data and might fail to generalize when given new data. Random Forests control overfitting by averaging multiple trees.
Pros. Random Forest helps us understand which features are most indicative of fake reviews. It can handle various user-related features and effectively incorporates diverse spatiotemporal features (geographic patterns, IP address, timing patterns).
Cons. The algorithm is less interpretable than simpler models and requires more computational power and memory.
Gradient Boosting Machines (GBM)
An ensemble technique trains several models (typically, decision trees) sequentially, each correcting the errors of its predecessor and iteratively improving predictive accuracy. Imagine trying to guess something and learning from each mistake you make, improving your guesses step by step. That’s what GBM does.
Pros. GBM's biggest advantages are improved prediction accuracy and the ability to handle complex data. It can become really good at spotting subtle and hard-to-detect patterns.
Cons. Due to its sequential nature, it’s slower to train and takes more computing resources compared to simpler algorithms.
Recurrent Neural Networks (RNNs)
This type of deep learning model can retain information from previous inputs through its internal memory. RNNs use a feedback loop to process new inputs alongside past data, allowing it to consider the entire input sequence.
RNNs are highly effective for handling sequential data (where the order of data elements matters). It’s often used for time series forecasting, speech recognition, and different natural language processing tasks. An ensemble of an RNN and a long short- term memory (LSTM) model, which is a type of RNN designed to handle longer dependencies, showed the ability to detect fake online ratings with the precision of about 94 percent.
Pros. RNNs, and especially LSTM models, can understand context and capture complex patterns and dependencies.
Cons. The method requires significant computational resources for training. Compared to traditional methods, it is more complex to implement and interpret. RNNs are prone to overfitting, especially when dealing with small datasets.
Fakespot and others: 5 tools for fake review detection
If your plans do not include building your machine-learning model for fake review detection, you can use one of the ready-made tools available on the market.
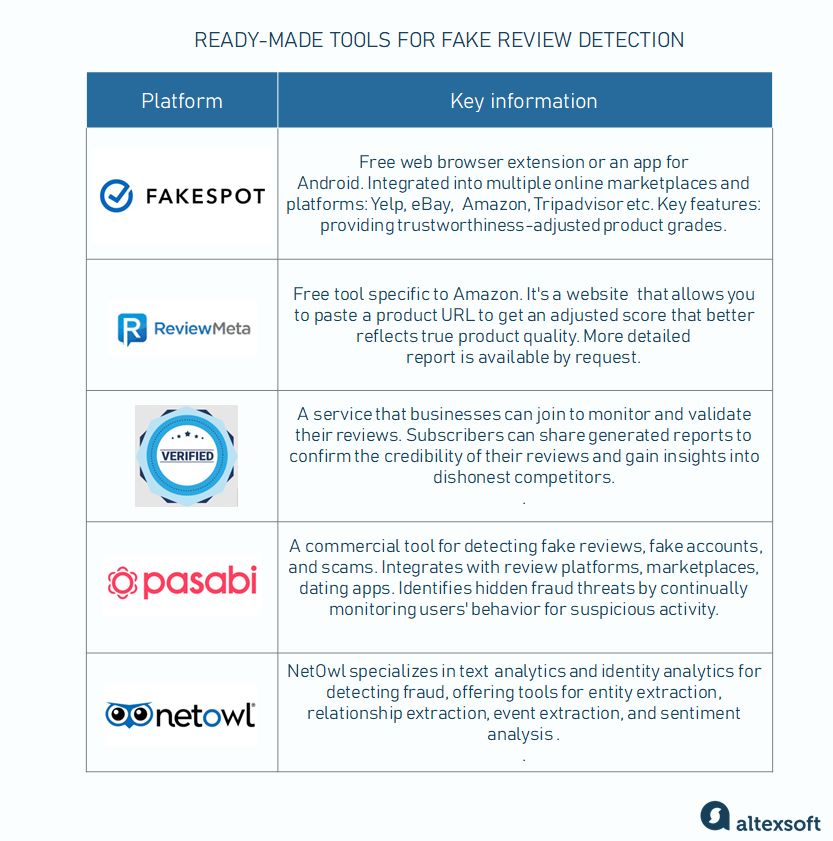
Tools for fake review detection
Fakespot is an easy-to-use, free web browser extension (or an app for Android). It analyzes review legitimacy and seller history to weed out fake reviews. Its key feature is to provide trustworthiness-adjusted product grades and review grades (levels from A to F). It is integrated into multiple online marketplaces and platforms: Yelp, eBay, Walmart, Amazon, Sephora, Flipkart, Home Depot, Steam, and Trip Advisor. But Mozilla, which owns Fakespot, plans to expand the list of platforms to integrate.
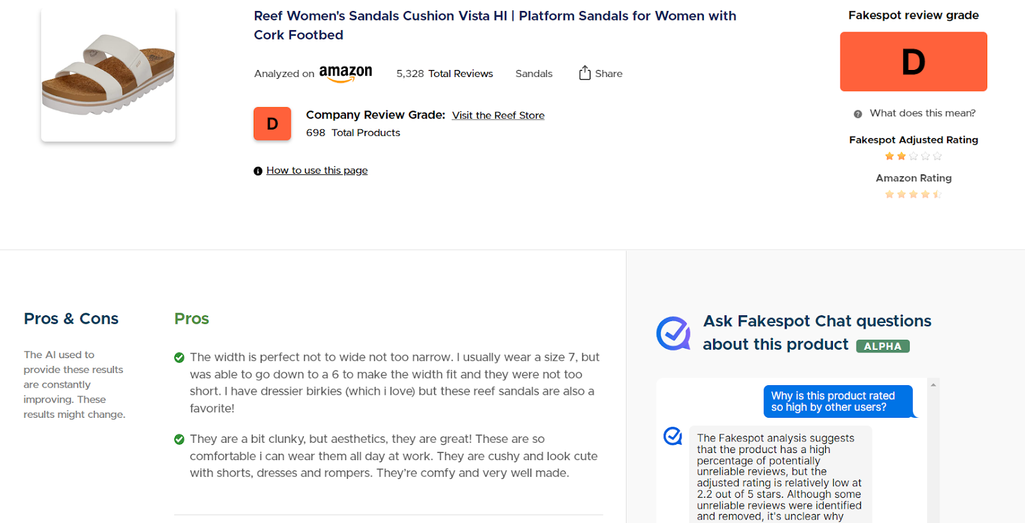
According to the report, the FakeSpot engine discovered that only 56 percent of the reviews for this product are reliable, and adjusted the rating accordingly. Source: Amazon
Besides getting an “approved” label, which boosts customers' trust, you can use Fakespot insights to help refine your product quality and customer service by addressing genuine concerns.
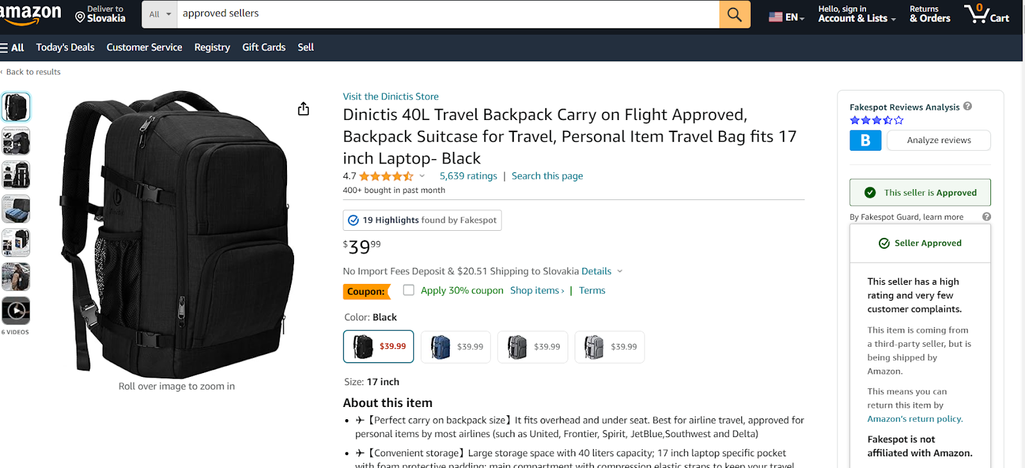
Fakespot also labels “approved” (reliable) sellers. Source: Amazon
ReviewMeta is a free tool somewhat similar to Fakespot but specific to Amazon. Its website allows you to paste a product URL to get a detailed analysis and an adjusted score that better reflects true product quality. If you wish, you can receive an even more detailed report by e-mail.
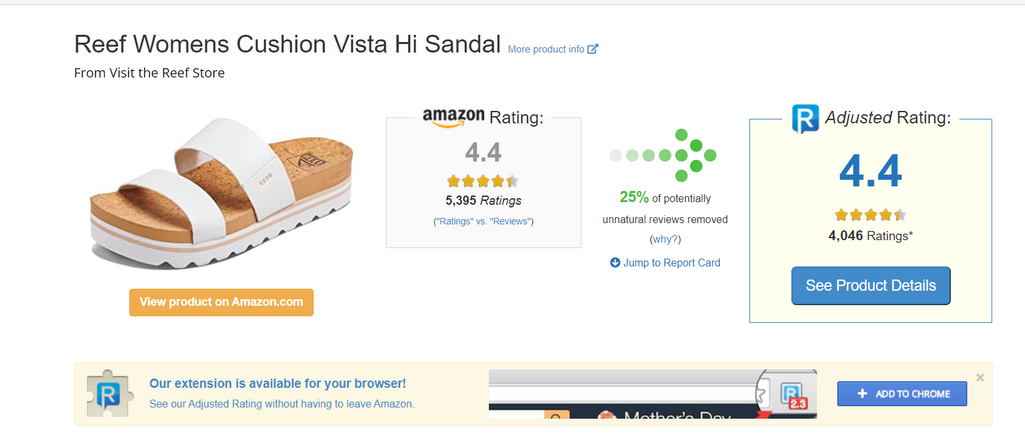
As you can see, ReviewMeta did not downgrade the original rating of this product on Amazon, although FakeSpot rated it skeptically. Source: RevieMeta
Transparency Company provides a subscription service to businesses for monitoring and validating their reviews. It integrates with various systems to ensure authenticity. Subscribers can share generated reports to confirm the credibility of their reviews and gain insights into dishonest competitors.
Pasabi is a commercial tool for detecting fake reviews, fake accounts, and scams. Although no such tools publicize their methods to prevent scammers from adapting to them, Pasabi claims it doesn't just detect individual fakes but entire fraud broker networks by checking the behavior and activity of sellers across multiple platforms. “Our software analyzes your data against our repository of known bad actors created from multiple data sources,” the website promises.
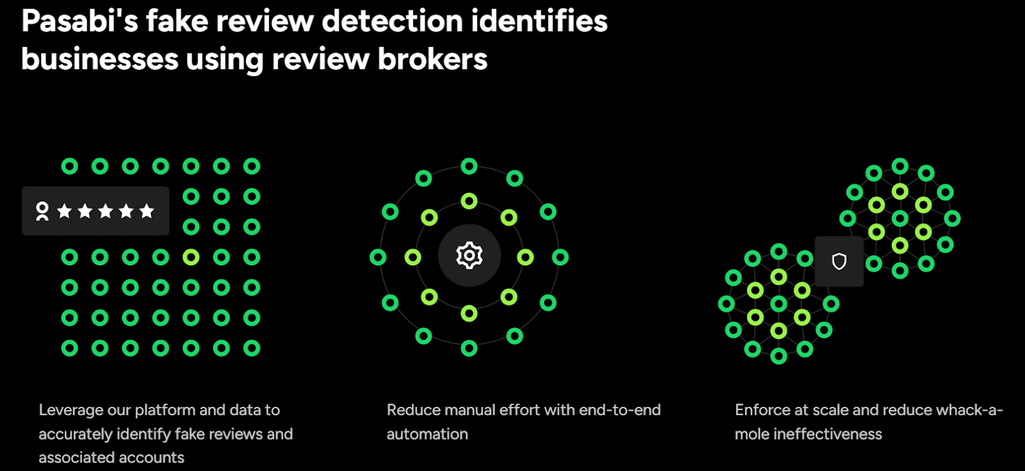
Pasabi detects fraud broker networks by checking the behavior of sellers across multiple platforms. Source: Pasabi
NetOwl is not explicitly designed as a tool for fake review detection, but it provides several functionalities that can be useful in this context. NetOwl specializes in text and identity analytics for detecting fraud, offering tools for entity extraction, relationship extraction, event extraction, and sentiment analysis.
Whatever option you choose, remember that the tool must be integrated with your website. Perhaps some of these tools are already pre-connected with your CRM; if not, you’ll need a custom integration via API.
Tech giants battle against fake reviews: real-life examples
Almost any major platform that relies on user-generated content takes the problem of bogus reviews seriously and applies AI algorithms to eliminate spam and false feedback.
Fake Amazon reviews
Amazon's marketplace is the hardest hit by fake reviews. A study conducted in 2023 using Fakespot found that about 43 percent of comments on the platform were deceptive.
For years, the eCommerce giant has been combating false feedback.
We use machine learning to look for suspicious accounts, to track the relationships between a purchasing account that's leaving a review and someone selling that product
As a result, the platform blocked more than 250 million suspected fake reviews from the store in 2023. The company also reported spotting more than 23,000 social media groups, with nearly 50 million members engaged in writing fake reviews on Amazon.
Fake Google reviews
Last year, Google launched a new method to detect and remove deceptive reviews. The algorithm identified suspicious activities of scam networks, which promised high-paying online tasks for a fee, leading to a surge in fake reviews. Investigators analyzed merchant reports and scammer contacts, identifying patterns, such as identical reviews across multiple business pages and sudden fluctuations in ratings.
Then, Google refined its algorithms to target affected business categories. Eventually, the company removed more than 170 million fake reviews in 2023, a 45 percent increase from 2022. More than 12 million phony business profiles were also removed or blocked.
Fake Yelp reviews
Yelp’s filtering algorithm predicts whether a review is solicited or biased based on data from reviews, reviewers, and businesses. The algorithm flags suspicious inputs, and human moderators check flagged content and investigate fraudulent activity.
Since 2012, Yelp has placed more than 4,900 consumer alerts on business pages to warn consumers when we find evidence of extreme attempts to manipulate a business’s ratings and reviews
The company uses different alerts for various types of fraudulent activity. For example, if there's evidence that someone has offered cash or other incentives in exchange for a review, a Compensated Activity Alert is displayed.
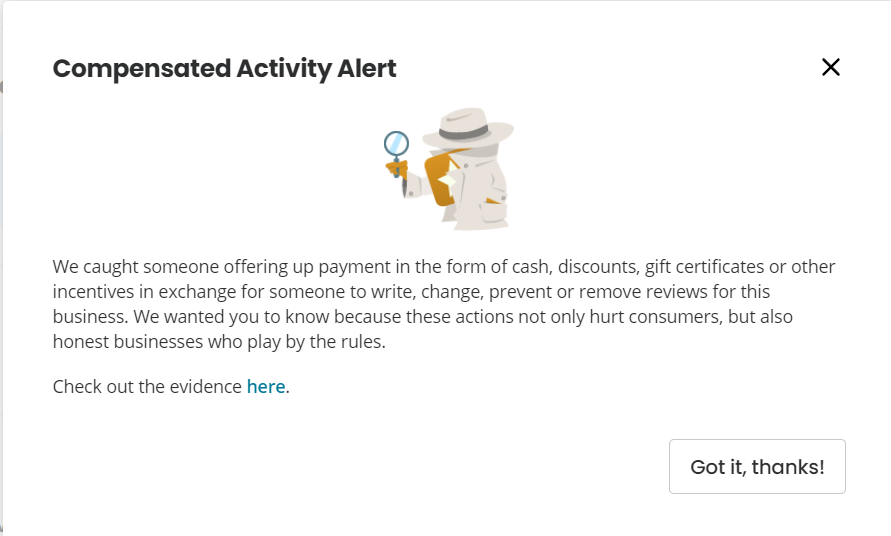
Compensation activity alert by Yelp
A Suspicious Review Activity Alert is shown if the filtering system notices many reviews from a single IP address or reviews from users who may be connected to a fraudulent group. In 2023, for user convenience, the company created a separate page that collected information about all the businesses and products that had been defamed.
Travel platforms fake reviews
Travel industry giants Booking.com and Expedia.com use a special approach, which supposedly filter out bad actors. The invitation to express an opinion about a particular hotel is only received by actual guests and only within a month after the stay is over.
However, this filter is not enough because even a guest with real experience can write a review for money received from an unscrupulous hotel. Therefore, in 2022, travel companies Booking.com, Expedia Group, and Tripadvisor established a coalition to fight fake reviews. Amazon, job and recruitment site Glassdoor, and business reviews site Trustpilot joined the alliance. The participants agreed to share content and information and to create common standards for fake review detection.
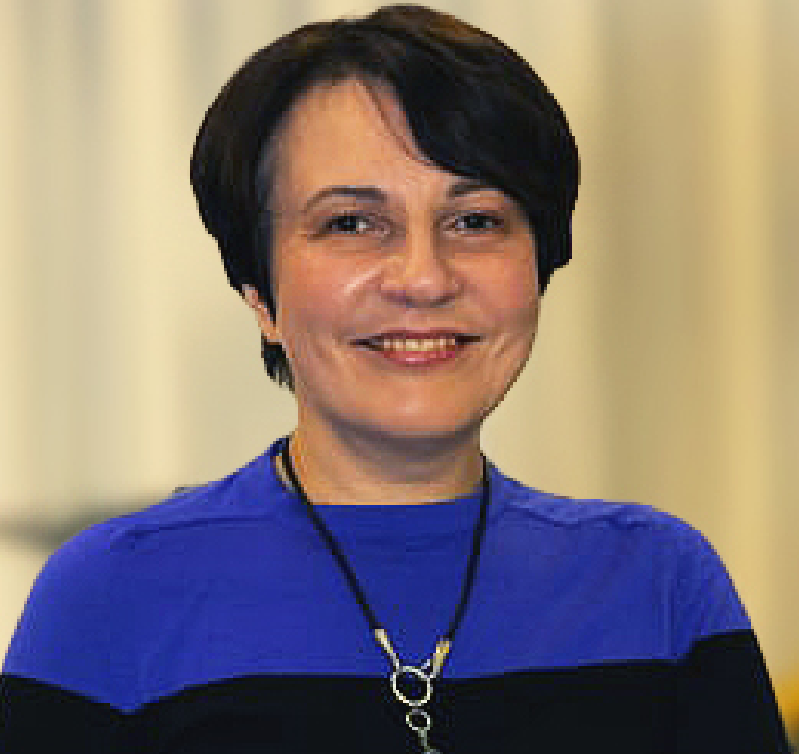
Olga is a tech journalist at AltexSoft, specializing in travel technologies. With over 25 years of experience in journalism, she began her career writing travel articles for glossy magazines before advancing to editor-in-chief of a specialized media outlet focused on science and travel. Her diverse background also includes roles as a QA specialist and tech writer.
Want to write an article for our blog? Read our requirements and guidelines to become a contributor.