Worse still, things reshaping customer intentions happen quite unexpectedly – from a competitor’s shop opening next door to the global lockdown due to the COVID-19 pandemic. Or consider the teenage climate activist Greta Thunberg. Her refusal to fly for environmental reasons kick-started the “flight shame” movement, which caused a five-percent decrease in air passenger numbers in Sweden.
There is no magic wand to predict scenarios like the “Thunberg effect”. But there are technologies to improve the accuracy of demand forecasting. Honestly, it will never be 100 percent precise, yet it can be precise enough to help you achieve your business goals.
In this article, we will look at the capabilities of advanced forecasting methods and outline their current limitations.
What is demand forecasting?
Demand forecasting is the estimation of a probable future demand for a product or service. The term is often used interchangeably with demand planning and demand sensing, but there’s a difference between the three. Let’s clear it up.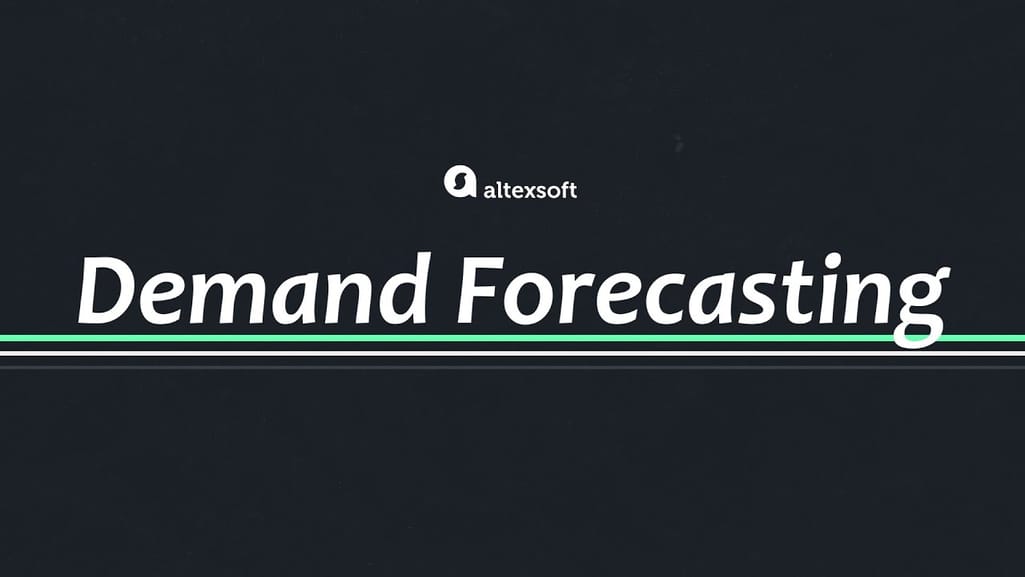
Watch our video for a quick overview of demand forecasting strategies
Demand planning — understanding market needs
Demand planning is a broader process that begins with forecasting but is not limited to it. According to the Institute of Business Forecasting and Planning (IBF), it applies “forecasts and experience to estimate demand for various items at various points in the supply chain.” In addition to making estimations, demand planners take part in inventory optimization, ensure the availability of products needed, and monitor the difference between forecasts and actual sales.
Demand planning serves as the starting point for many other activities, such as warehousing, shipping, price forecasting, financial planning, and, especially, supply planning that aims at fulfilling the demand and requires data on the anticipated needs of customers.
Demand sensing – creating short-term predictions
A relatively new concept in the planning process, demand sensing is a forecasting method that employs advanced analytical techniques to capture real-time fluctuations in purchase behavior. The technology can be of great help for companies, operating in fast-changing markets.
Demand sensing solutions extract daily data from POS systems, warehouses, and external sources to detect an increase or decrease in sales by comparison with historical patterns. The system automatically evaluates the significance of each divergence, analyzes influence factors, and offers adjustments to short-term plans.
Adopting demand sensing reportedly reduces near-time forecast errors by 30 to 40 percent. It empowers companies to rapidly address sudden changes in customer needs and facilitates building a data-driven supply chain. Of course, you can’t make all decisions based on this technique alone, as it doesn’t work for mid- or long-term planning. But it may serve as a valuable complement to traditional forecasting methods.
A demand sensing software dashboard, capturing a change in demand in the short term, and showing factors that cause the fluctuation. Source: E2Open
Here, again, we return to forecasting. Getting as close to reality as possible is the key to improving efficiency across the entire supply chain. How do you reach the uppermost accuracy possible? The answer depends on business type, available resources, and objectives. Let’s compare the existing options: traditional statistical forecasting and machine learning algorithms.
Traditional statistical forecasting — good for stable markets, ill-disposed to changes
Traditional statistical methods (TSM) have been here for ages and remain a staple of forecasting processes. The only difference if compared with the previous century is that all calculations are performed automatically, by modern software. For example, you can create time-series forecasts for sales and trends in Excel.
Data sources. To predict the future, statistics utilizes data from the past. That’s why statistical forecasting is often called historical. The common recommendation is collecting data on sales for at least two years.
Why use it. Traditional forecasting is still the most popular approach to predict sales, and for a reason. As a rule, demand planning solutions based on statistical techniques seamlessly integrate with Excel and existing Enterprise Resource Planning (ERP) systems without requiring additional tech expertise. The most advanced systems can consider seasonality and market trends as well as apply numerous methods to finetune results.
Things to consider. An important prerequisite of statistical forecasting accuracy is stability. We assume that history repeats itself: Situations that occurred two or three years ago will reoccur. Which is far from being true. Flawless in an ideal world, statistical methods often fail to foresee illogical alterations in customer preferences or predict when market saturation will occur.
A statistical forecasting software dashboard. Source: Streamline
Best fit. All in all, automated statistical forecasting offers a satisfying level of accuracy for:
- mid- to long-term planning,
- well-established products, that enjoy stable demand, and
- predicting total demand rather than sales of separate stock-keeping units (SKUs).
Does it make business sense to invest in more sophisticated technologies? We’ll try to clear things up in the next section.
Machine learning for demand planning — advanced accuracy at the price of added complexity
Increased computer power on the one hand and increased demand volatility on the other created prerequisites for wider use of machine learning (ML) to design predictions. Say, demand sensing that we mentioned above solely relies on ML techniques to generate short-term predictions in response to diverse market changes. ML also drives predictive analytics beyond just estimating demand. It combines historical and current data to generate insights in trends and custom behavior under certain conditions.
Data sources. Built upon statistical models, machine learning utilizes additional internal and external sources of information to make more accurate, data-driven predictions. ML engines can work with both structured and unstructured data including
- past financial and sales reports (historical data),
- marketing polls,
- macroeconomic indicators,
- social media signals (retweets, shares, spikes in followers),
- weather forecasts,
- news about local events,
- competitors activity, and more.
Data sources for demand forecasting with machine learning. Source: IBF (Institute of Business Forecasting and Planning)
Why use it. Machine learning applies complex mathematical algorithms to automatically recognize patterns, capture demand signals, and spot complicated relationships in large datasets. Apart from analyzing huge volumes of information, smart systems continuously retrain models, adapting them to changing conditions thus addressing volatility. These capabilities enable ML-based software to produce more accurate and reliable forecasts in complex scenarios.
What does more accurate really mean? Companies that added machine learning to their existing systems report an increase of 5 to 15 percent in forecast reliability (up to 85 and even 95 percent). In addition to this, your team gets rid of time-consuming manual adjustments and recalibrations.
Things to consider. To take advantage of the machine learning solution, you need sufficient processing power and really large batches of high-quality data. Otherwise, the system won’t be able to learn and generate valuable predictions.
Also, bear in mind the additional complexity in terms of software maintenance and result interpretation. While ML mechanisms come to conclusions without human intervention, it’s up to a live tech expert to determine what features should be fed to the model, which of them have the largest impact on the output, and why the model generates a certain prediction. Check our detailed article about roles in a data science team to get a picture of which specialists have to be involved.
Best fit. The list of situations in which machine learning definitely works better than traditional statistics includes:
- short- to mid-term planning,
- volatile demand patterns,
- fast changing environment, and
- new product launches.
Comparison between traditional and machine learning approaches to demand forecasting
As you can see, employing machine learning comes with some tradeoffs. Depending on the planning horizon, data availability, and task complexity, you can use a combination of different statistical and ML solutions.
Whichever methods you choose, you’ll need specialized software that can help you with your predictions.
Demand forecasting software: how to choose
Today, there are a lot of solutions on the market that can support your demand planning activities. They have different capabilities, and the choice depends on your business needs. Here are several things you should consider if you're thinking of acquiring an off-the-shelf tool.
Functionality
Obviously, the first thing you have to look at is whether it fits your business requirements. Depending on your industry and business model, you might need
- short-term or long-term predictions (or both);
- demand forecasting for new products;
- multitiered planning for different regions, channels, and product groups;
- price elasticity estimation;
- multidimensional modeling and comparisons of what-if scenarios (for promotions, price changes, market fluctuations, display variations, assortment changes, etc.);
- accounting for halo effects and product cannibalization (when demand fluctuations of one product impact complementary or competitive items);
- granular dashboards and reports, and so on.
How promotion influences demand. Source: Relex
Compatibility with your business tools
It’s crucial to connect your internal systems (like ERP or sales management software) to a demand forecasting solution to enable data sharing, collecting complete historic information, and building demand trends. Besides, smooth integrations with your inventory management system or warehouse management system (WMS) will allow you to streamline procurement and capacity management.
Many vendors of demand forecasting software offer out-of-the-box integrations with the most popular ERP providers, Excel, and other business tools, so check if the chosen provider can assist you with system connections. If not, you’ll have to engage IT specialists to build internal integrations.
Tech support and training
Logically connected to the previous recommendation, make sure the software provider offers all the necessary support during and after implementation. Such tools aren’t something you download and just start using – they need a lot of data for analysis that has to be imported properly. Also, remember that you’ll have to conduct sufficient training for your staff.
Data sources and external factors
Depending on your industry, you might need to consider external factors to increase the accuracy of your predictions, e.g., weather, macroeconomic trends, and others. Contact your provider to find out which data sources they use.
Typically, the more data you have, the more impacting factors would be considered, and the more accurate your forecasts will be. But building such complex, custom analytical infrastructure requires investment and engagement of ML engineers, data scientists, and other specialists. However, it pays off. Let’s talk about some real-world examples of successful ML-based demand forecasting.
When machine learning works best for demand planning: successful use cases
Highly variable environment, dozens of factors driving buying behaviors, many types of data involved — all these often make demand planning too complex to be successfully performed with simple tools. So, big companies choose to invest in smart technologies to optimize their inventory management.
Nestlé implements demand-driven forecasting
Nestlé used to create 80 percent of their forecasts with human intervention. But they wanted to better understand their customer motives. SAS forecasting and analytics technology allowed them to sense and analyze demand signals associated with sales promotions, price, advertising, in-store merchandising, and economic factors.
Charles Chase, an Industry consultant, reports, “Today, 80 percent of Nestlé's forecasts are driven right out of the solution with no human judgment at all. …Every one percent improvement in forecast accuracy translated into a two percent reduction in inventory safety stock. They were eventually able to take out anywhere between 14- 20 percent of their inventory safety stock, reduce it and still meet consumer demand with this improved forecasting capability. If you have US $100 million in inventory that’s a US $20 million reduction.”
PUMA adopts an integrated approach to inventory management
PUMA experienced losses and had a gap between supply and demand due to disconnected business systems and fragmented tools. After implementing a comprehensive solution with data management, forecasting, and simulation capabilities, they managed to fix and standardize their planning and analytics processes. As a result, they streamlined procurement, mitigated shortages and residual stock, and gained fuller visibility into both external market conditions and internal operations.
UK hospitals reduce waste from blood overstocks with ML
The significant complexity of supply chain, short-term demand spikes, and the high cost of errors (with human lives at stake) prompted the Blood and Transport department of the UK’s National Health System (NHS) to transfer from spreadsheets and manual databases to ML-fueled planning system with enhanced predictive capabilities. It allowed hospitals to reduce waste from blood overstocks by 30 percent without any drop in service quality and enabled rapid responding to potential shortages. “If there’s no yogurt on the supermarket shelf — well, that’s unfortunate. If there’s no blood in the hospital, the consequences are very different,” an NHS executive explained as the reason to invest heavily in the advanced solution.
How to approach demand forecasting?
If you want to implement any kind of demand forecasting solution to enhance your supply chain and planning operations, there are several important considerations.
Buy or build?
As we said, the answer depends on your needs and resources.
Use a demand forecasting module. The easiest (not the cheapest) thing to do is get comprehensive business software such as ERP or WMS that already has an in-built demand forecasting module and manage all your business operations from a single system.
Pros and cons: you don’t need to build internal integrations, but the functionality might be limited and hard to customize.
Buy a separate tool. If you’re big enough to track and forecast demand, but still not ready for a full-blown ERP, get a specialized solution that fits your needs.
Pros and cons: It’s cheaper and you’re more flexible with choosing the functionality, but you’ll have to build bespoke integrations.
Build a custom system. If you have unique business needs and want to have a system tailored for them, it will require custom development.
Pros and cons: Maximum customization and accuracy, but takes a lot of time and investment.
Test the waters with ML
While adoption of machine learning tools can somewhat narrow the gap between anticipation and reality, it doesn’t mean that every company should immediately jump to complex intelligent technology. You can start with small enhancements to your existing system that will address those problems that are difficult to solve by traditional methods. For example, use a machine learning module to make data-driven changes in planning for the short term and leave long-term forecasting to old-school statistics.
Remember about cost-effectiveness
Sometimes it turns out that dealing with forecast errors (such as excessive stock) is cheaper than fine-tuning your ML models to obtain maximum accuracy. Don’t get carried away with perfectionism; better calculate the ROI and think if further investment is worth it.
Besides, sometimes forecasts are erroneous because of random or completely unpredictable factors, so any efforts to increase prediction accuracy are pointless.
Who could've predicted those notorious toilet paper shortages? Source: New Harbor Consultants
Integrations and testing are key to success
Some 20 years ago, the retail giant Nike invested $400 million in their supply chain software and demand planning system. However, instead of having the right inventory to fulfill customer demand, they ended up losing $100 million because the predictions were wrong. It happened because the system wasn’t tested sufficiently. It turned out that it had some bugs and wasn’t properly integrated with data sources.
The lesson learned: Build seamless integrations to establish smooth data exchange and give sufficient attention to your testing activities to make sure your system functions properly and forecasting results are reliable.
Short-term forecasts are typically more accurate
It’s simply easier to predict what happens in a day or two than trying to imagine what life will be like in a year. Starting from simple factors like weather and ending with ever-changing customer preferences and other unstable market and macroeconomic conditions, the shorter the time frame the more accurate the prediction is likely to be.
Human brains still matter
Forecasting demand is a challenging task, and it still has much room for improvement. Upland, the U.S. provider of business management software, claims that sales forecasts are less than 75% accurate and calculates that the US economy spends around $50 billion on predictions that are pretty much worthless. Sounds scary, doesn’t it?
However smart your forecasting solution is, the key decisions still rest with human capital. You need industry specialists to define which factors should be considered in your predictive models. Human logic is still required to evaluate the relevance of outcomes produced by digital brains and to make final conclusions based on common sense and deep domain expertise.
That’s why even ML-powered demand planning systems often include a collaborative platform that allows for engaging different specialists in a forecasting process. Only by taking the best of what both artificial and human intelligence offer can you see and plan a better future for your business.