We discussed with data science specialists and AI startuppers how airline industry players use cognitive technologies to reach new heights. But first, consider checking our article dedicated to data science, AI, ML, and other modern buzzwords to learn how they differ.
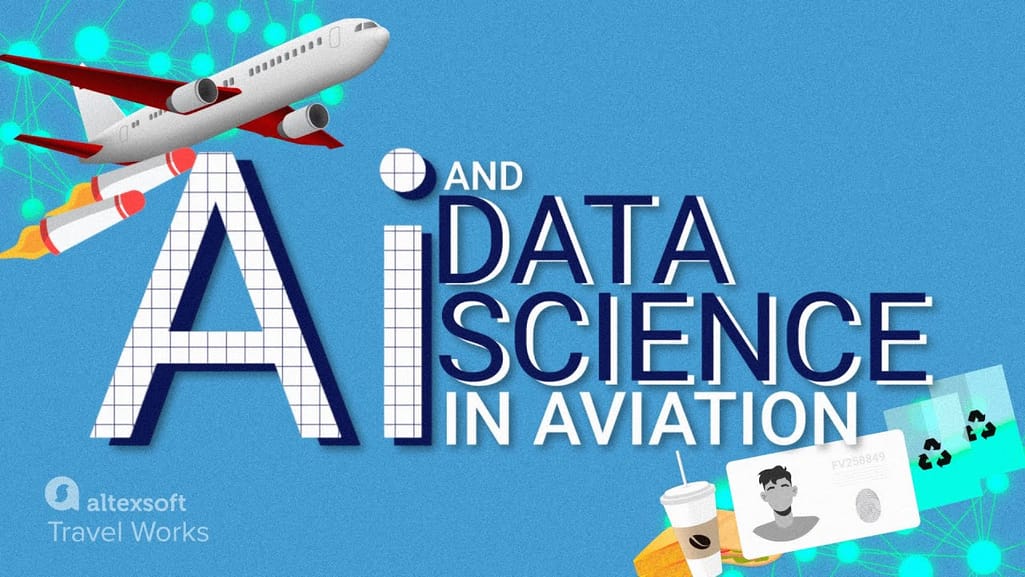
Watch a short video about AI use cases in aviation
The main applications of AI and data science in the airline industry
Artificial intelligence and its cognitive technologies that make a sense of data can streamline and automate analytics, machinery maintenance, customer service, as well as many other internal processes and tasks. So, AI technologies are useful for various aspects of airline operation management.
How airlines leverage AI
1. Revenue management
Revenue management (RM) is the application of data and analytics aimed at defining how to sell a product to those who need it, at a reasonable cost at the right time and using the right channel.
It’s based on the idea that customers perceive product value differently, so the price they are ready to pay for it depends on target groups they belong to and purchase time.
Revenue management specialists make good use of AI to define destinations and adjust prices for specific markets, find efficient distribution channels, and manage seats to keep the airline simultaneously competitive and customer-friendly.
Data scientist Konstantin Vandyshev, who worked at Transavia's Revenue Management department, stresses that data science disciplines come in handy for achieving revenue management tasks.
Demanded flight routes. While RM is about finding the best way to sell a product or service, carriers use AI to answer one of the key questions: where to fly? “To define air routes, specialists have to analyze data and make decisions based on the insights. When researching a demand for a destination among different customer groups, they can rely on such data sources as search history and macroeconomic factors (e.g. GDP),” says Konstantin.
RM has industry-specific standards that specialists must use to define willingness to pay.
Willingness to pay. Collecting and crunching data about customers, airlines understand passengers’ tastes and behavior well enough to offer them transportation options they prefer and, more important, are ready to spend money on. So, revenue managers start from measuring willingness to pay (WTP).
Willingness to pay reveals "when" a customer is likely to pay "a maximum price" for a product or service, explains the data scientist. “It’s assumed that customers are ready to pay more when there is less time before departure time. And society finds this pricing fair. WTP in the airline industry, therefore, depends on the day before departure (DBD). In practice, specialists define median WTP — a price that 50 percent of customers would like to pay for a ticket on a specific DBD. Such WTP is equivalent to price elasticity (the number of passengers that would buy a ticket if a price drops by a certain percent) with some assumptions between market demand and supply.”
This metric is connected to dynamic pricing — the practice of pricing a product based on a specific customer’s willingness to pay. The calculation of WTP requires selecting data correctly. Revenue management can combine similar markets and, alternatively, distinguish high and low seasons, as well as holidays and weekends.
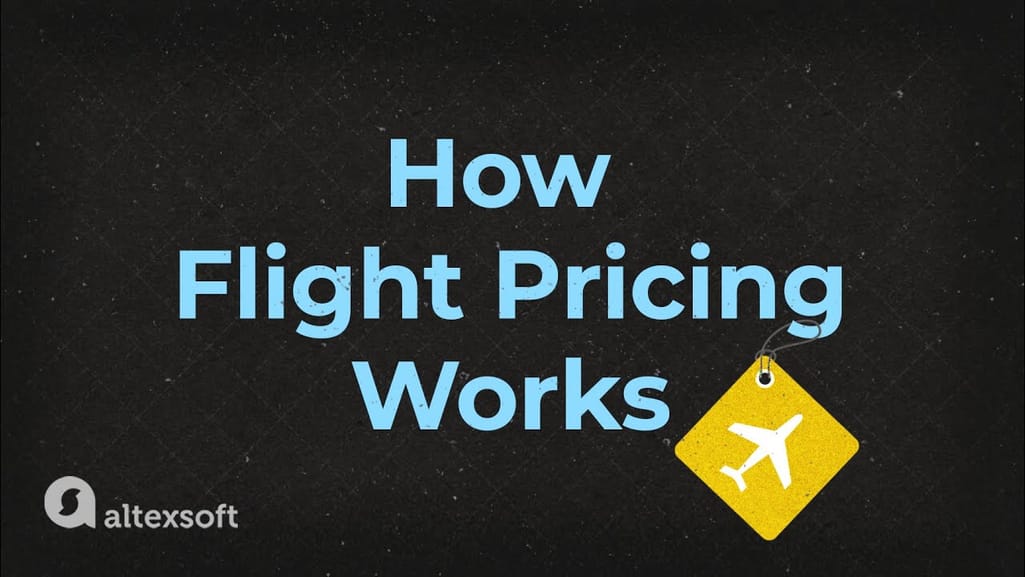
Watch how dynamic pricing works in the airline industry
“Approaches to this type of statistical analysis were developed nearly 10 years ago. These days, it’s easier to conduct research and present its results thanks to the development of data science and visualization capabilities. Considering that each case is unique, it’s very important to choose the right amount of data to extract insights from," concludes Konstantin.
Expected marginal seat revenue (EMSR). This optimization model is calculated after WTP is defined. The metric can be perceived as the expected value of the current seat and entails allocating a seat to a specific fare class (FC). Data scientists measure EMSR by multiplying sales profit by the probability of selling an additional (marginal) seat belonging to the particular FC. “The moment comes when sales probability of a higher-fare ticket is so low that the expected revenue in a lower fare class will be bigger. So, knowing these probabilities you can determine the fare-class allocation for each day before departure,” adds Konstantin.
In a best-case scenario, specialists have to know the sell-up probabilities for different fare classes and days before departure to determine WTP and EMSR accurately, says this expert. The sell-up probability discloses whether a customer is likely to buy a higher-fare ticket if their request is denied. Clustering of flights according to destinations and flight dates is required. The revenue management team also carries out a clickstream analysis to know how many customers saw a web page showing a specific price. Airlines use historical sales data when determining willingness to pay and expected marginal seat revenue.
Ancillary price optimization. This is another approach designed to increase airline revenues through analytics-driven pricing. It allows data scientists to learn about a traveler's tendency to buy ancillaries like baggage. Specialists define in which markets and on what days people are likely to pay more to check their bags. “For example, if I book tickets for three people with a child, then I’m ready to pay X euros more than if I flew alone somewhere on a weekend,” explains Konstantin Vandyshev.
Revenue management starts with analyzing demanded flight routes
2. Air safety and airplane maintenance
Airlines literally bear high costs due to delays and cancellations that includes expenses on maintenance and compensations to travelers stuck in airports. With nearly 30 percent of the total delay time caused by unplanned maintenance, predictive analytics applied to fleet technical support is a reasonable solution.
Carriers deploy predictive maintenance solutions to better manage data from aircraft health monitoring sensors. Usually, these systems are compatible with both desktop and mobile devices, granting technicians access to real-time and historical data from any location. Knowing an aircraft’s current technical condition through alerts, notifications, and reports, employees can spot issues pointing at possible malfunction and replace parts proactively. Executives and team leads, in turn, can receive updates on maintenance operations, get data on tool and part inventory, and expenses via dashboards.
With applied predictive maintenance, an airline can reduce expenses connected with expedited transportation of parts, overtime compensation for crews, and unplanned maintenance. If a technical problem did occur, maintenance teams could react to it faster with workflow organization software.
Shane Ballman, former manager of Maintenance Systems & Technology at AirTran Airways and CEO of AI startup SynapseMX, Inc, came up with a platform that utilizes historical and real-time data to help maintenance crews make technical decisions faster.
“We automate the routine and mundane using the company's workflows, elevating things to the right person at the right time when a human's touch is required,” says Shane.
The SynapseMX software analyzes data and metadata regarding detected maintenance activity. It helps engineers quickly evaluate a situation, for instance, to find out if this failure happened for the first time; if not, what can be done to fix it and how much time did it take before. Employees can also specify if there are spare parts available or a conflicting workload. “Then we evaluate for business rules — who cares, and under what conditions do they care? Should this trigger a new workflow? Update metrics? Send notifications?
Our AI is able to provide recommendations, in real-time, from the technicians in the field to the logistics team that supports them. The end result is a maintenance organization that reacts intelligently to current conditions,” concludes Ballman.
Predictive maintenance is great, but it doesn’t replace essential aircraft inspections. AI solutions can be of assistance here as well. For example, Donecle offers an automated drone and image analysis algorithms to detect defects on the aircraft, making inspections way faster and more efficient.
3. Feedback analysis
Air travel can be stressful even for frequent, experienced travelers whose passports are running out of clean pages. They have to do so many tasks like checking bags or finding a gate before getting themselves into a plane seat and taking a selfie!
In this regard, airlines that learn about pain points of airport and flight experience through data analysis can improve customer service. Using AI for feedback analysis and market research allows airlines to make informed decisions and meet customers’ expectations, agrees founder and CEO of PureStrategy Inc. Briana Brownell.
“AI systems can quickly allow airlines to determine if there is an opportunity to positively intervene in the customer journey and turn a poor experience into a delightful one. It also allows companies to react faster in a synchronized, aligned way that is on-brand and consistent with the business’s values.”
PureStrategy introduces a platform for business analytics called the Automated Neural Intelligence Engine (ANIE). The engine’s functionality includes data review, categorization, visualization, and sentiment analysis. So, the engine does a lot of manual and time-consuming work with information allowing humans to concentrate on more complex tasks.
“We deal with customer feedback and voice of the customer data throughout all areas of the organization. Then, we link this data to internal operational metrics as well as external industry metrics,” specifies data scientist. Briana emphasizes the growing relevance of natural language understanding technology in processing and analysis of customer experience data as it allows for exploring the customer journey in their own words.
ANIE can be used to make sure whether it’s easy for customers to find, book, and pay for flights. “Ultimately we want to understand the ways in which an airline can delight a customer as well as where there is friction in the customer journey — and figure out how to fix it,” concludes Briana Brownell.
4. Messaging automation
When a disruption such as a flight delay or baggage loss occurs, travelers get nervous. And if customers don’t get a response or explanation of a problem from an airline representative in a timely manner, they likely won’t choose this airline for their next trip. The speed of response to customer queries matters as much as actual steps that are taken to solve an issue.
AI software speeds up and simplifies customer service employees’ workflows by using algorithms for processing natural language or unstructured text. For example, such systems can classify emails and extract information from them.
Another way to automate and improve customer service is chatbot development. Today, many airlines enhance their customer support with AI-powered chatbots that help passengers with booking and managing flights, tracking baggage, answering questions, and providing other types of assistance.
5. Crew management
Imagine a scheduling department that has to assign crews to each of thousands of flights operated every day. That’s a lot of work. Specialists consider a myriad of factors: flight route, crew member licensing and qualification, aircraft type and fuel usage, work regulations, vacations and days off to approve conflict-free schedules for pilots and flight attendants. Besides those are airplane maintenance schedules, training requirements like the pairing of senior crew members with junior ones, and government regulations that have to be taken into account.
“Crew management is a complex task due to many legal constraints. For instance, if staff belong to a trade union, limitations include an allowed number of flight hours and days off, as well as reimbursement in case of a labor law violation,” сlarifies data scientist Konstantin Vandyshev.
Of course, schedulers are not left to go it alone with big data generated by airlines (like, maintenance and passenger data, or data from onboard sensors.) Employees rely on software that integrates data from various sources, allowing them to get a full picture of daily operations. Using uncovered insights, they are able to make an optimal schedule in terms of working time, crew qualification, aircraft utilization, and expenses.
In other words, such software integrates predictive models with an airline operations management system.
Some crew management solutions allow addressing fatigue risk that pilots are in danger of due to a constant change of time zones, long duty days, scheduling changes, and other “pleasures” of working in the airline industry. For example, developers of the Crew Rostering solution from Jeppesen started integrating bio-mathematical models of fatigue into flight crew scheduling software. Their goal is to provide schedulers with the ability to rely on data about predicted fatigue to reduce risks in the planning phase.
At the end of the day, it’s all about safely transporting people from point A to point B.
6. Fuel efficiency optimization
Global aviation produces nearly 2 percent of anthropogenic carbon dioxide (CO2) emissions. That’s why aircraft manufacturers and carriers strive to improve their fuel efficiency. Well, it’s not only ecological but also financial concerns that drive airline industry players to use technology to reduce carbon emissions. According to Investopedia, airlines spend 10 to 12 percent of their operating costs on fuel.
Airlines use AI systems with built-in machine learning algorithms to collect and analyze flight data regarding each route distance and altitudes, aircraft type and weight, weather, etc. Based on findings from data, systems estimate the optimal amount of fuel needed for a flight.
7. In-flight sales and food supply
Eating a sandwich with a cup of coffee while enjoying the passing clouds and a bright blue sky is how many of us imagine an airplane journey.
Meanwhile, supply management specialists define how many snacks and drinks they must onboard without being wasteful. AI is here to help, too.
“If a low-cost airline sells food on board, it has to know how to predict the amount of food it has to buy for a specific flight,” says Konstantin. “While food isn’t expensive, every cargo load costs money. In addition, airlines usually throw away a lot of sandwiches at the end of the day. Companies that will be the first to solve this problem can make a good commercial use case out of it.”
8. Fraud detection
IATA estimates that airlines lose around 1 billion dollars annually due to payment fraud. There are numerous fraudulent schemes, including paying with stolen cards, claiming reimbursement for “lost” baggage, creating fake online travel agencies, and so on.
Fraud cases are becoming more sophisticated but so are fraud management techniques. In addition to enhancing customer authentication and payment security, airlines implement advanced, ML-based analytics software to process data from multiple sources and detect fraudulent transactions. Check our article dedicated to fraud detection to learn more about such systems and the main approaches to fraud management.
Besides direct fraud, airlines experience losses due to so-called revenue leakage. Its sources include no-shows and cancelations, fare discrepancies, tariff abuse, and so on. Modern analytical solutions help identify potential revenue leakage cases and take preventive measures.
9. In-airport self-service
The COVID-19 pandemic caused an unprecedented rise of various contactless technologies. Self-service check-in kiosks systems were just the first step of automating the passenger journey. Today, airlines and airports implement end-to-end solutions across multiple terminals, facilitating and expediting passenger flow from check-in to boarding.
For example, smart systems such as Fly to Gate by Thales utilize biometrics technology and document scanners to recognize and verify passengers at security checks. Meanwhile, baggage identification solutions like BagsID that are capable of detecting individual bags by their unique physical characteristics enable contactless baggage handling. All these technologies reduce passenger check-in time and improve customer experience.
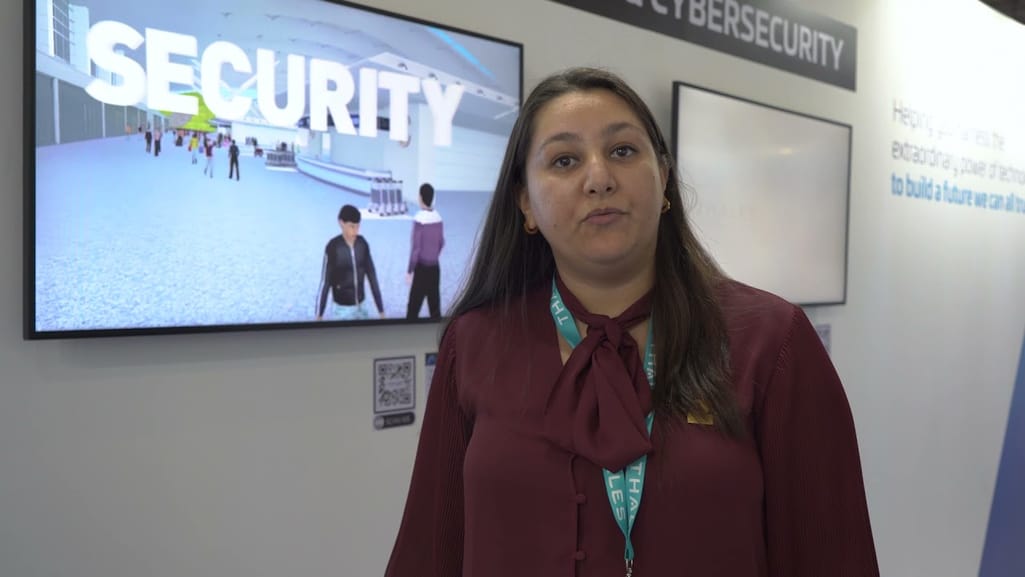
Fly to Gate solution overview
10. Flight management and autonomous taxi, take-off, and landing in the future
Self-driving, or autonomous vehicles are no longer sci-fi. Such innovators as Waymo and Google introduced driverless cars years ago, forecasting a bright and promising future with multiple application areas. The idea seems simple. The vehicle has to be equipped with numerous cameras and “taught” to interpret road conditions in real time and react accordingly. In reality, complex ML algorithms are necessary to bring this idea to life.
In 2018, Airbus started its Autonomous Taxi, Take-Off and Landing (ATTOL) project that was successfully concluded in 2020. Airbus demonstrated a completely automated, vision-based take-off and landing, controlled by on-board image recognition technology. While this research is still in the experimental stage, its success holds potential for enhancing future aircraft operations.
As of today, such innovative AI platforms as Flyways make use of real-time data received from multiple sensors and external sources to support flight management and optimize the flight path. That allows pilots and dispatchers to make better decisions during the flight, find optimal routes, and reduce delays caused by bad weather conditions, turbulence, etc.
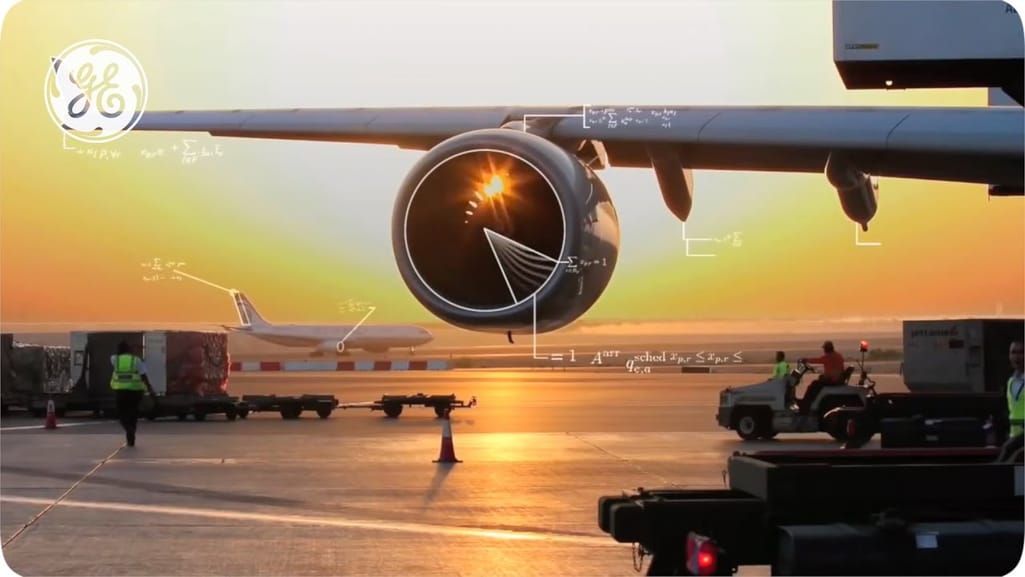
GE Aviation is the provider of digital solutions that use advanced analytics techniques to reduce delays and optimize aircraft operations
Airlines using AI to improve operations
As you can see, there are a lot of ways airlines can make use of AI and data science to automate their operations, reduce expenses, and increase customer satisfaction. Here, we’ll describe only a few real-world examples.
Delta: self-service for better customer experience and predictive aircraft maintenance
Delta, one of the world’s largest global airlines, leverages AI to optimize operations and costs, as well as innovate customer service at every stage of a trip. In 2019, it ranked second in the Travel category of the world’s most innovative companies by Fast Company. Here are some of its AI-based projects.
Airport journey enhancement. In early 2021, Delta launched the facial recognition technology to identify passengers at the airport touchpoints. To participate, you only need a passport number, a Fly Delta app, and a TSA PreCheck membership. When at the airport, you just look at the camera, scan your face to use your digital identity in place of a physical ID and boarding pass -- and voila, a complete touchless experience is yours.
In October 2021, Delta expanded its innovation program, introducing bag-drop technologies powered by biometric data.
Delta offers a complete hands-free passenger journey
In-flight experience improvement. In January 2020, Delta announced the AI-driven system that supports operational decisions in critical conditions like bad weather. It used a digital twin technology to create a full-scale simulation environment that processes tons of data points, predicts possible outcomes, and equips decision makers with the most effective options to handle disruptions and ensure safety.
Predictive maintenance. While an airline has no control over such disruptions as bad weather or air traffic control problems, it’s responsible for delays or cancellations due to technical issues. Delta is no stranger to predictive fleet maintenance. In 2018, Delta partnered with Airbus to make use of the Skywise Core Platform and Skywise Predictive Maintenance App in an effort to improve aircraft reliability. The investment paid off. The number of maintenance-related cancelations dropped from more than 5,600 in 2010 to just 55 in 2018.
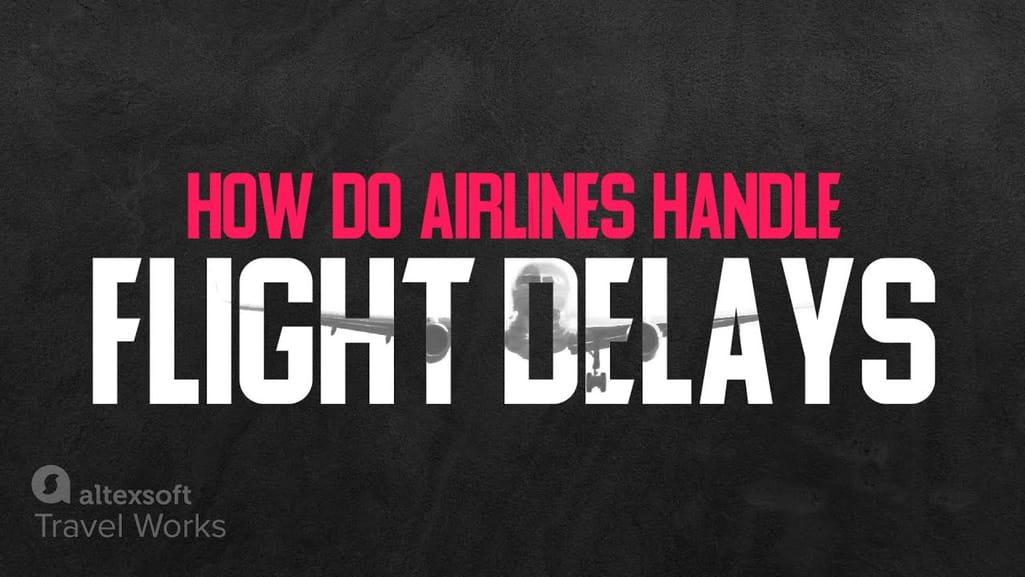
Find out why flights get delayed and how delays are handled
Air France: reducing CO2 emissions
Air France is one of the biggest and oldest airlines in Europe. In 2021 it was included in the list of the best airlines in the world by Skytrax rating for the quality of its airport and onboard product and staff service.
Prefacing IATA’s Net-Zero Carbon Emissions resolution, Air France implemented the specialized Sky Breath software as part of its Air France Horizon 2030 program along with other sustainability-focused initiatives. This AI platform collects data from the flight, performs in-depth analytics, and helps identify fuel-saving opportunities and increase efficiency.
Other airlines that leverage AI to optimize fuel consumption include Norwegian, Malaysia Airlines, Atlas, and more.
You can read more about sustainability in travel and carbon offsetting in aviation in our dedicated articles.
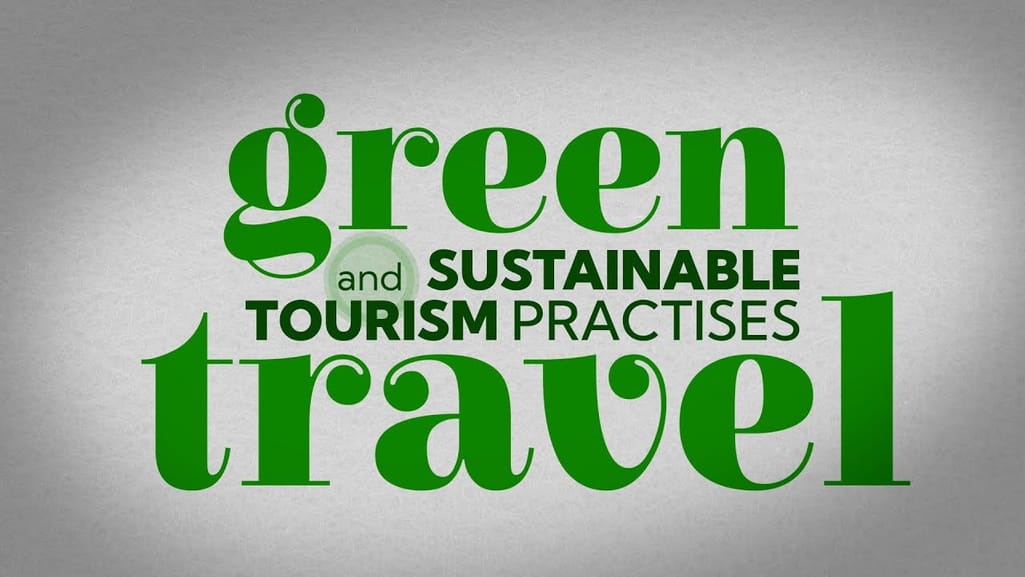
Green travel overview
Alaska Airlines: intelligent route planning
For many years in a row, Alaska Airlines has been one of the leading carriers for customer satisfaction, according to such industry-recognized rating platforms as J.D. Power North America Airline Satisfaction and the American Customer Satisfaction Index (ACSI). It has also been one of innovation pioneers since the 90s, starting with selling tickets online, introducing self-service kiosks, and leveraging GPS technology. And it keeps leading the way.
In May 2021, Alaska Airlines signed the contract for the use of the Flyways AI platform. It’s a flight monitoring and routing tool that assists dispatchers in making informed decisions and planning new, efficient routes. The system creates data-based predictions and provides recommendations on flight operations and routing. Within the six-months trial period, Flyways enabled the airline to reduce miles, save 480,000 gallons of fuel, and avoid creating 4,600 tons of carbon emissions.
Southwest Airlines: optimization and excellent customer experience with big data analysis
Dallas-based Southwest Airlines is the largest low-cost carrier in the world and the largest domestic airline in the US. The airline calls itself a “Customer Service company that happens to fly airplanes,” and it seems that this strategy is working. Southwest was ranked the best US airline according to TripAdvisor’s 2020 Travelers Choice Awards.
Great flight experience is the evidence that customer service quality meets traveler expectations. To maintain the fleet in good technical condition, control the work of each department, and know how a traveler feels about their trip, airlines strive to build their own data strategy.
Listening to what your customers say and improving operations based on their suggestions are fundamental steps to achieving high-level customer service. That’s exactly what Southwest does in its Listening Center that was opened in 2014. Forty experts from Customer Relations, Marketing, and Communication departments monitor social media feeds to enable the airline to solve emerging issues as rapidly as possible.
Advanced software captures related keywords across the web, letting team members track sentiment on social media about Southwest, its competitors and the airline industry as a whole, analyze the most popular industry-related topics discussed on social platforms, and follow news from traditional media. Insights from social media help the airline stay current with trends and provide customers with personalized offers.
The Listening Center specialists explore data from various resources 24/7. Photo source: Southwest Airlines
Southwest also uses a flight analytics system by GE Aviation to improve fuel consumption for its fleet of more than 700 Boeing 737s. The cloud-based software that runs on the Industrial Internet enables specialists to collect and analyze data generated by aircraft during a flight to plan the optimal amount of fuel.
The future of AI in the airline industry
Today, AI makes it possible to enhance customer experience with automation and self-service solutions, optimize employee workflow, and ensure higher air safety with predictive and prescriptive aircraft maintenance. It also allows airlines to make informed decisions about pricing and market positioning through the smart use of data.
“It’s worth mentioning various stochastic optimization tasks in regards to potential use cases of AI in the airline industry. Eventually, data science is applied to operations optimization, but carries great hope for the development of new technologies,” notes Konstantin Vandyshev.
Briana Brownell also admits AI’s key role for operations optimization. “I see many opportunities! For instance, to optimize operations including adding, changing or removing routes, setting flight times, pricing, and product offerings. Ultimately, success is driven by having a deep understanding of different customer segments and where new market opportunities exist,” concludes this data scientist.
We’ve told you about a few airlines and their data science initiatives. Feel free to comment how your business uses AI in the comment section below.