You most likely believe that you can extract value from your business' data. However, PwC report has shown that only 4 percent of surveyed companies have been able to seize the information advantage. The rest of the organizations – the so-called misguided majority – not only have lacked the required skills and technical capabilities to successfully utilize the data, but also failed to create an organizational culture that contributes to growth.
No matter how many staff data scientists you have or which database you use, if you want to use your data effectively, you need a business-aligned culture that supports how your data is stored and the way people access and work with it.
An analytics – data-driven – culture is based on the belief that each one of your business decisions should rely on data.
What attributes define a corporate culture analytics-driven?
Therefore, the problem of implementing an analytics culture is not always in a company's technical realm, but rather in its human-resource realm, the individuals who work for the company. The changes that come with a new approach to doing business must be addressed first and foremost from the employee side.
There are several typical steps that allow companies to successfully start a data science adoption. Let’s examine them.
Connect the dots. List all the ways your data is already being used or the sources where your employees obtain it. Is each department using the same tools introduced by top management or are their methods inconsistent and spontaneous?
Assess existing decision-making. Define the impact data is already having on your decision-making progress. Is it used to evaluate the results and make intuitive decisions from there? Or maybe your marketing department has already been incorporating social media analytics to make future decisions?
Set data priorities. Compare the existing data use to the areas you want to implement analytics in the future. Map out the specific steps that will lead to complete data absorption.
You may already have useful metrics, not only for each functional unit, but also your staff members. Google, for instance, uses data about team productivity and from employee surveys to identify the traits common to the best managers and their biggest challenges.
Clarify the vision. Don't invest in analytics only as a response to the demand for digital transformation. Even if your employees know about the importance of analytics, they may have no clear understanding of which specific business questions it will help them answer in their job. Ultimately, let your team know which part of your business strategy data will support.
Allocate the roles. Take all of the foreground tasks where you want to actively use analytics and describe how and to what extent each functional unit will have to work on them. Who will be responsible for defining the most valuable data and distributing it to other departments? What share of your data initiative depends on each employee in each department? Make sure that you’ve mapped out the route for data across your organization from top to bottom.
A Salesforce survey discovered that the most popular business use cases for data today are driving operational efficiencies and facilitating growth.
Collect data. First, make an effort to track and gather all data you have now and use it to your advantage with the methods that are already available to you. In the huge pool of data, be sure you know which pieces of it should be measured to succeed at your goals.
Standardize records. Apply standards to your information and pick the one that will be responsible for key business decisions. On a journey to universally coordinated and accessible data, it’s important to have a set of norms applied to each piece of information. This entails standard data formats and the ways it is recorded.
According to Salesforce, high-performing companies are two times more likely than underperformers to empower half of their employee base to use analytics tools. If you are looking to rely on data in as many decisions as possible, you need every decision-maker to freely use dashboards, reports, and spreadsheets, and overall – be data literate.
Data literacy can encompass a wide range of skills, and most people don’t need to be able to program in R. Unless you’re using some machine learning as a service solutions (MLaaS) to help staff operate data with minimum data wisdom, make sure that each department member is trained to understand, summarize, and visualize data freely.
Describe a training plan for all levels of staff. Break down your data literacy strategy into levels for different types of employees and departments. Who will only be required to understand spreadsheet data and use the tools, and which departments require a more extensive knowledge and expertise? Then, initiate data science training or assign a more experienced employee to mentor others. Make sure you serve as a good example by equipping your meetings with great visualization and metrics usage.
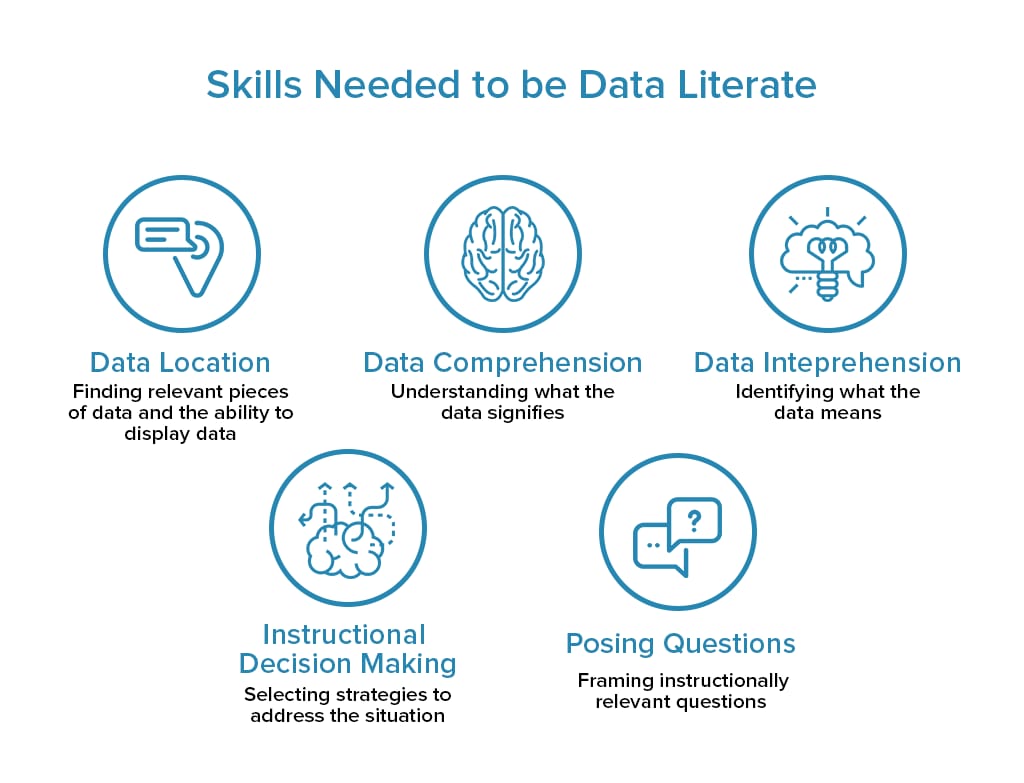
“Evangelize data competency,” shares Ryan Farley, co-founder of LawnStarter that fosters a successful analytics-driven culture. “For a while, we'd do trainings at night on analytics tools so that people who don't regularly look at data can gain an appreciation for the power of data. In addition, many of these people started doing their own data analysis in their role.”
This way, even the departments that don’t have a data scientist can be data-driven. However, while the goal is to allow non-technical users to operate data freely, it doesn’t mean that you can get along without a proper data science team if you plan to scale for predictive and prescriptive analytics. Someone has to prepare the datasets, ensure that info is gathered from call centers and sales departments, and create working systems.
Eliminate different versions of truth. Data standardization that we talked above becomes more challenging once it covers multiple departments that use different tracking tools. If a company doesn’t follow a standardized process, units start to collect data and metrics from different sources, sometimes utilizing incorrect or low-quality information, which results in one of the biggest enemies of a data-driven culture – different versions of truth.
Democratize access to data. In an analytics-driven culture, there’s no “our” or “their” data. If one type of data proves to be useful in one department, other teams should be informed. Make sure that no data stays in one department as a power tool but rather gets unreservedly shared whenever employees need it.
“Empower the team to do analysis,” suggests Ellie Mirman, CMO for marketing intelligence provider Crayon. “Specifically in orgs where data may be centralized, it's still important to give data access to every employee and reward and encourage analysis. If you hoard data and analysis in a single department, the data-driven culture will never form across the business.”
For instance, a machine learning-driven delivery service DoorDash allows each staff member to access datasets at any time and makes sure that even non-technical employees can use data effectively. Upon breaking information silos, you will eliminate multiple versions of truth and develop coherency across departments in the way they implement your data strategy.
Outline KPIs. The data-driven approach implies setting clear KPIs that are comprehensive and indicative of whether a manager or a department merely does the job. If there are other implicit factors left to evaluate performance, then you have a problem. These “other factors” bias your performance understanding and leave you unaware of the real results. So, get rid of them and focus on KPIs.
Your team also needs to see what’s being evaluated and how these indicators are calculated. Create the spreadsheets where your employees will measure and track their results, and make a habit of regularly reviewing their KPIs.
Mathias Golombek, CTO at EXASOL, recommends: “Make sure that results of the data-driven decisions can be qualified. There is no more powerful way to convince people and keep improving things than proving the results by hard facts and numbers.”
Automate KPIs tracking. Once you’re sure about your KPIs, the tracking can be automated. This fits well for a flat organizational structure that we’ll talk about in a minute. Higher management doesn’t have to track KPIs regularly for possibly hundreds of departments on a subordinate level. Instead, the system can send notifications only if something goes wrong in one of the departments and KPIs aren’t matched.
There are several aspects of creating a culture where one person’s opinion doesn’t dominate over analytics results. Demystifying the data and bringing data literacy that we discussed earlier will help your HiPPOs see data not as a threat but as a tool that grants confidence and facilitates the decision-making process.
Reconcile HiPPOs with data. Ensure that data doesn’t exist to discredit past decisions but to replace the intuition-based aspect in the new ones. You can’t ignore your employee's experience but embrace healthy discussions in your operations and use data to make sure they’re unbiased.
Introduce A/B testing. You can adopt a culture of objective experimentation. Test each decision and determine the success metrics. After you have the results of both data- and opinion-based decisions, you can prevent HiPPOs from choosing unproductive ideas.
A great example from Kellogg’s vice president of business intelligence Rick Davies: “For example, when the temperature drops, more soup is sold. Kellogg sells crackers, which go well with soup, so the hypothesis is that more crackers will be sold when it gets colder outside. But, if you don't have the data to prove that, you cannot make business decisions based on that hypothesis.”
Reconsider your structure. You can eliminate those bottlenecks by transforming your company’s structure and enabling horizontal coordination. Data will help you calculate the performance KPIs and control the changes in every division without human integration. Now you can remove redundant hierarchical links and save time executing tasks.
Start with available machine learning APIs. Many off-the-shelf solutions today allow newcomers understand the potential revenue impact of specific products and predict customers’ spending habits in their specific fields. If you just start, you can apply data to existing MLaaS platforms like Microsoft Azure, Google Prediction API, or Amazon Machine Learning, which will allow you to build the foundation of overall data science intelligence in your company. With the limited number of potential data scientists to hire, you can still unleash the power of predictive analytics.
Scale your predictive analytics capabilities. MLaaS platforms are good for training homegrown experts in data science. But they are limited in terms of further scaling for much more complicated operations. These need data engineers to set infrastructure and continuous data collection. Once you have a qualified team – either homegrown or hired – you can scale for predictive and prescriptive analytics. The latter can help you automate decision-making.
It requires spreading data across all your functional units, having effective conversations, and being able to understand how and why analytics can bring a transformative change. By making sure that data flows without restraint inside your organization, and staff members are engaged and trusting, not only will you revolutionize the way your information is operated but will also cultivate a new era of improved performance and cross-divisional communication.
What hurdles have you faced on your journey to a data-driven culture? Do you have any tips on stimulating the transformative lifestyle in your business? We’d like to hear from you in the comments below.
No matter how many staff data scientists you have or which database you use, if you want to use your data effectively, you need a business-aligned culture that supports how your data is stored and the way people access and work with it.
An analytics – data-driven – culture is based on the belief that each one of your business decisions should rely on data.
What attributes define a corporate culture analytics-driven?
- Opinions and assumptions can be changed based on data.
- The company embraces improvements suggested by data.
- Employees can manage data independently.
- Analytics is a part of everyone’s job.
- Data is organized, accessible, and of a high quality.
- Each opinion is supported by numbers and data.
- Every employee can access data that relates to them.
- Reports are replaced with interactive analytics tools.
Therefore, the problem of implementing an analytics culture is not always in a company's technical realm, but rather in its human-resource realm, the individuals who work for the company. The changes that come with a new approach to doing business must be addressed first and foremost from the employee side.
There are several typical steps that allow companies to successfully start a data science adoption. Let’s examine them.
Assess your current data culture
To define your main analytics goals, be aware of the data situation your organization already has and learn to work in the existing environment. By making note of the current situation, you’ll be able to identify the most urgent goals and act upon them.Connect the dots. List all the ways your data is already being used or the sources where your employees obtain it. Is each department using the same tools introduced by top management or are their methods inconsistent and spontaneous?
Assess existing decision-making. Define the impact data is already having on your decision-making progress. Is it used to evaluate the results and make intuitive decisions from there? Or maybe your marketing department has already been incorporating social media analytics to make future decisions?
Set data priorities. Compare the existing data use to the areas you want to implement analytics in the future. Map out the specific steps that will lead to complete data absorption.
You may already have useful metrics, not only for each functional unit, but also your staff members. Google, for instance, uses data about team productivity and from employee surveys to identify the traits common to the best managers and their biggest challenges.
Make analytics a strategic priority
Businesses often start analytics initiatives without having a realistic picture of how analytics might fit into their existing processes and operations. Think of it like starting to pack your bags before even deciding where and when you’re traveling. Such behavior, introduced by top management, results in a lack of understanding and engagement from the rest of the team. That’s why no matter which technologies and talented data scientists you employ, you won’t magically make your organization data-driven and data-motivated.Clarify the vision. Don't invest in analytics only as a response to the demand for digital transformation. Even if your employees know about the importance of analytics, they may have no clear understanding of which specific business questions it will help them answer in their job. Ultimately, let your team know which part of your business strategy data will support.
Allocate the roles. Take all of the foreground tasks where you want to actively use analytics and describe how and to what extent each functional unit will have to work on them. Who will be responsible for defining the most valuable data and distributing it to other departments? What share of your data initiative depends on each employee in each department? Make sure that you’ve mapped out the route for data across your organization from top to bottom.
A Salesforce survey discovered that the most popular business use cases for data today are driving operational efficiencies and facilitating growth.
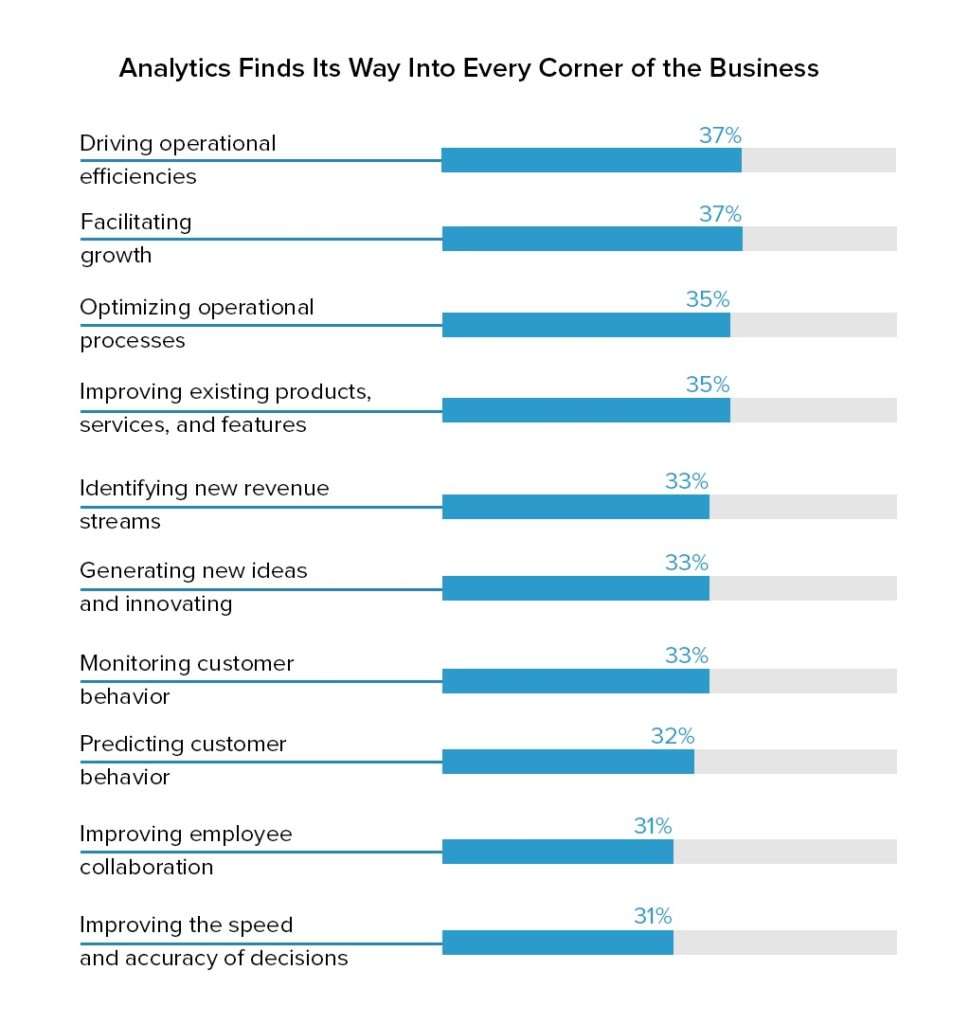
Source: Salesforce
Health insurance provider Cigna has been strategically using analytics to understand customer needs. Cigna’s Scott Filiault, vice president of information management and analytics, notes “Our goal is to broaden our understanding of consumers and what their needs are. We’re also focusing on how we evolve our brand, and how we attract and retain targeted customers by applying analytics to improve the consumer experience and value of our solutions.”Manage your existing data
At the early stages, you’re still dealing with descriptive analytics, which provides the insight on the past decisions, just like your regular sales or customer reports.Collect data. First, make an effort to track and gather all data you have now and use it to your advantage with the methods that are already available to you. In the huge pool of data, be sure you know which pieces of it should be measured to succeed at your goals.
Standardize records. Apply standards to your information and pick the one that will be responsible for key business decisions. On a journey to universally coordinated and accessible data, it’s important to have a set of norms applied to each piece of information. This entails standard data formats and the ways it is recorded.
Eliminate the knowledge gap for non-technical users
The problem with analytics tools and techniques is that they aren’t accessible to the decision makers. Many legacy companies don’t have custom and well-designed software to unify and visualize data. Data tracking tools may be highly technical and often require a statistics Ph.D. to operate, which is partly why the data science talent is in demand across all industries. The added layer of complexity leaves business leaders unable to get the most valuable answers when they need it.According to Salesforce, high-performing companies are two times more likely than underperformers to empower half of their employee base to use analytics tools. If you are looking to rely on data in as many decisions as possible, you need every decision-maker to freely use dashboards, reports, and spreadsheets, and overall – be data literate.
Data literacy can encompass a wide range of skills, and most people don’t need to be able to program in R. Unless you’re using some machine learning as a service solutions (MLaaS) to help staff operate data with minimum data wisdom, make sure that each department member is trained to understand, summarize, and visualize data freely.
Describe a training plan for all levels of staff. Break down your data literacy strategy into levels for different types of employees and departments. Who will only be required to understand spreadsheet data and use the tools, and which departments require a more extensive knowledge and expertise? Then, initiate data science training or assign a more experienced employee to mentor others. Make sure you serve as a good example by equipping your meetings with great visualization and metrics usage.
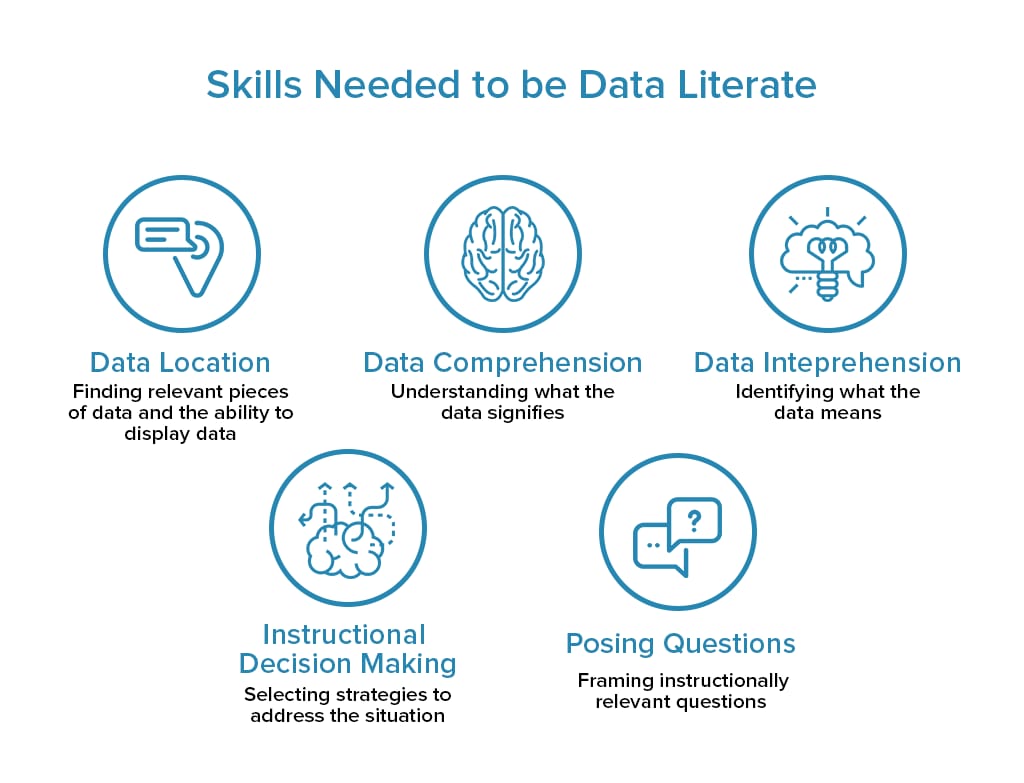
“Evangelize data competency,” shares Ryan Farley, co-founder of LawnStarter that fosters a successful analytics-driven culture. “For a while, we'd do trainings at night on analytics tools so that people who don't regularly look at data can gain an appreciation for the power of data. In addition, many of these people started doing their own data analysis in their role.”
This way, even the departments that don’t have a data scientist can be data-driven. However, while the goal is to allow non-technical users to operate data freely, it doesn’t mean that you can get along without a proper data science team if you plan to scale for predictive and prescriptive analytics. Someone has to prepare the datasets, ensure that info is gathered from call centers and sales departments, and create working systems.
Break down the silos
The siloed structure of departments is another barrier to building a data-driven organization. Divisions themselves may avoid sharing data collected inside, or access to data can be too complicated and overregulated. The EY’s survey found that 81 percent of companies agree that data should be at the heart of all decision-making but only 31 percent have significantly restructured their operations to do this.Eliminate different versions of truth. Data standardization that we talked above becomes more challenging once it covers multiple departments that use different tracking tools. If a company doesn’t follow a standardized process, units start to collect data and metrics from different sources, sometimes utilizing incorrect or low-quality information, which results in one of the biggest enemies of a data-driven culture – different versions of truth.
Democratize access to data. In an analytics-driven culture, there’s no “our” or “their” data. If one type of data proves to be useful in one department, other teams should be informed. Make sure that no data stays in one department as a power tool but rather gets unreservedly shared whenever employees need it.
“Empower the team to do analysis,” suggests Ellie Mirman, CMO for marketing intelligence provider Crayon. “Specifically in orgs where data may be centralized, it's still important to give data access to every employee and reward and encourage analysis. If you hoard data and analysis in a single department, the data-driven culture will never form across the business.”
For instance, a machine learning-driven delivery service DoorDash allows each staff member to access datasets at any time and makes sure that even non-technical employees can use data effectively. Upon breaking information silos, you will eliminate multiple versions of truth and develop coherency across departments in the way they implement your data strategy.
Clearly define KPIs and automate their tracking
In order to participate in creating a data-driven culture, your leaders need to know which metrics are important for the company and their smaller tasks. While nearly all companies have and track key performance indicators (KPIs), these figures may stay only as supportive metrics that don’t directly impact decisions.Outline KPIs. The data-driven approach implies setting clear KPIs that are comprehensive and indicative of whether a manager or a department merely does the job. If there are other implicit factors left to evaluate performance, then you have a problem. These “other factors” bias your performance understanding and leave you unaware of the real results. So, get rid of them and focus on KPIs.
Your team also needs to see what’s being evaluated and how these indicators are calculated. Create the spreadsheets where your employees will measure and track their results, and make a habit of regularly reviewing their KPIs.
Mathias Golombek, CTO at EXASOL, recommends: “Make sure that results of the data-driven decisions can be qualified. There is no more powerful way to convince people and keep improving things than proving the results by hard facts and numbers.”
Automate KPIs tracking. Once you’re sure about your KPIs, the tracking can be automated. This fits well for a flat organizational structure that we’ll talk about in a minute. Higher management doesn’t have to track KPIs regularly for possibly hundreds of departments on a subordinate level. Instead, the system can send notifications only if something goes wrong in one of the departments and KPIs aren’t matched.
Depersonalize decision making
Author and entrepreneur Avinash Kaushik has coined the term Highest Paid Person’s Opinion or HiPPO, that describes that one person existing in every organization with decades of experience and an excessive influence on the decision-making process. These people are experts in their fields, but often refuse to agree with data if it contradicts their opinion. Such guesswork which is based on intuition and gut feeling can slow down the business and starve the analytics culture you’re trying to nourish.There are several aspects of creating a culture where one person’s opinion doesn’t dominate over analytics results. Demystifying the data and bringing data literacy that we discussed earlier will help your HiPPOs see data not as a threat but as a tool that grants confidence and facilitates the decision-making process.
Reconcile HiPPOs with data. Ensure that data doesn’t exist to discredit past decisions but to replace the intuition-based aspect in the new ones. You can’t ignore your employee's experience but embrace healthy discussions in your operations and use data to make sure they’re unbiased.
Introduce A/B testing. You can adopt a culture of objective experimentation. Test each decision and determine the success metrics. After you have the results of both data- and opinion-based decisions, you can prevent HiPPOs from choosing unproductive ideas.
A great example from Kellogg’s vice president of business intelligence Rick Davies: “For example, when the temperature drops, more soup is sold. Kellogg sells crackers, which go well with soup, so the hypothesis is that more crackers will be sold when it gets colder outside. But, if you don't have the data to prove that, you cannot make business decisions based on that hypothesis.”
Use data to automate operational decisions
The over-complicated hierarchical structures with numerous vertical elements don’t allow for rapid decision-making and slows feedback from the bottom of the hierarchy. Having to pass several links that are often represented by HiPPOs makes it almost impossible to launch the data-driven culture simultaneously across the whole enterprise. The fuel company BP with operations in 72 countries struggled to execute new decisions between the numbers of structural layers and functions. Using data, they determined that the best decision makers had to get too many approvals from the regional heads and as a result, they centralized some operations, removed the middle management layer, and saved $3 billion in costs.Reconsider your structure. You can eliminate those bottlenecks by transforming your company’s structure and enabling horizontal coordination. Data will help you calculate the performance KPIs and control the changes in every division without human integration. Now you can remove redundant hierarchical links and save time executing tasks.
Start small with predictive analytics
As you scale up, you’ll be moving on to the second stage – obtaining the information from your data that will help you visualize the future outcome. Predictive analytics and the introduction of machine learning strategy can give you actionable insight and fill in the missing data using statistical models and algorithms. By capturing the relationship between many different factors and assessing the set of conditions, you will be able to reliably predict the outcome of your decision before making it.Start with available machine learning APIs. Many off-the-shelf solutions today allow newcomers understand the potential revenue impact of specific products and predict customers’ spending habits in their specific fields. If you just start, you can apply data to existing MLaaS platforms like Microsoft Azure, Google Prediction API, or Amazon Machine Learning, which will allow you to build the foundation of overall data science intelligence in your company. With the limited number of potential data scientists to hire, you can still unleash the power of predictive analytics.
Scale your predictive analytics capabilities. MLaaS platforms are good for training homegrown experts in data science. But they are limited in terms of further scaling for much more complicated operations. These need data engineers to set infrastructure and continuous data collection. Once you have a qualified team – either homegrown or hired – you can scale for predictive and prescriptive analytics. The latter can help you automate decision-making.
The big picture
Having a successful data initiative requires a serious cultural change.It requires spreading data across all your functional units, having effective conversations, and being able to understand how and why analytics can bring a transformative change. By making sure that data flows without restraint inside your organization, and staff members are engaged and trusting, not only will you revolutionize the way your information is operated but will also cultivate a new era of improved performance and cross-divisional communication.
What hurdles have you faced on your journey to a data-driven culture? Do you have any tips on stimulating the transformative lifestyle in your business? We’d like to hear from you in the comments below.
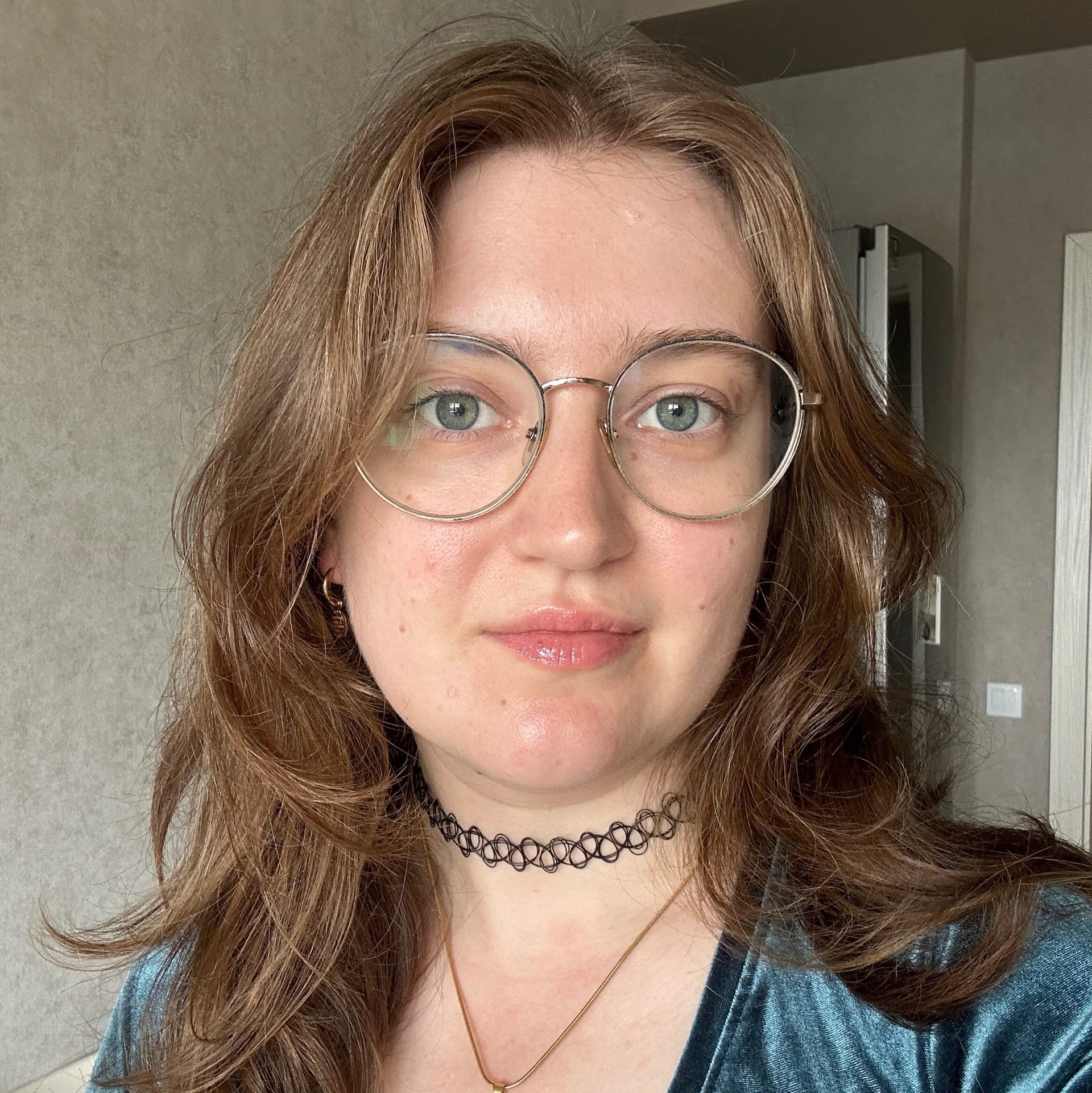
Maryna is a passionate writer with a talent for simplifying complex topics for readers of all backgrounds. With 7 years of experience writing about travel technology, she is well-versed in the field. Outside of her professional writing, she enjoys reading, video games, and fashion.
Want to write an article for our blog? Read our requirements and guidelines to become a contributor.