Revenue management (RM) is a set of procedures the travel industry implements to sell rooms, tickets, and services to the right people, at the right time, and for the right price.
This complex task embraces several components that are shaped by demand, market conditions, customer behavior, and demographics:
- customer segmentation
- demand forecasting
- pricing techniques
- yield management
While digital transformation effectively impacts all four, the one we’re spotlighting is pricing and the application of dynamic pricing techniques. Dynamic pricing allows hotels (or any other travel industry players, such as airlines and car rental companies) to adjust prices on the fly by constantly recalculating the demand/supply spectrum. Here's how, for instance, dynamic pricing works in airlines.
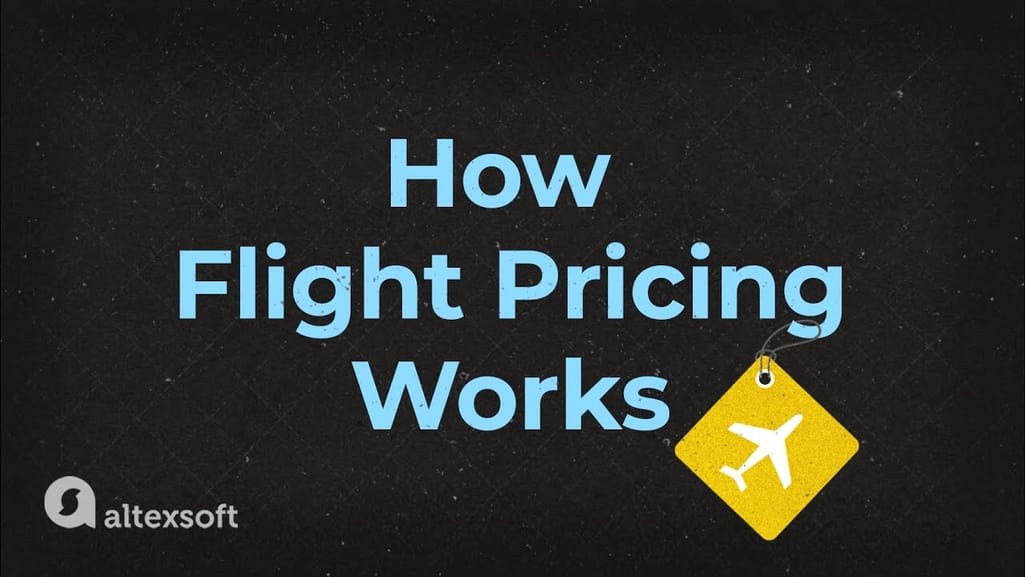
Similar to hotels, airlines have been using dynamic pricing for years
Dynamic pricing applied by hotels in only as old as the early part of this century, when such chains as Marriott, Hilton, and InterContinental implemented their first RM software systems. The Lodging Technology Study reveals that 60 percent of US hotels used dynamic pricing in 2015. However, according to a number of estimates, hospitality is lagging behind other travel industry subdivisions. For instance, airlines have been utilizing RM techniques over the last 30 years.
Source: The 2017 Smart Decision Guide to Hospitality Revenue Management
As time marches on, the revenue management digital revolution has yet to happen. The industry shift from simple legacy systems to smarter data-driven solutions is straggling. In this article, we’ll discuss novel RM approaches and show how data analysis allows for building precise and robust dynamic pricing applications.
Legacy vs next gen revenue management
Hotel legacy systems are often complicated and technically deficient. For instance, revenue managers must manually tweak prices, limiting the agility of the dynamic pricing approach. The problem extends to other operations as well. Guests may be segmented only by the purpose or length of travel. Demand forecasting may be based on simple methods like “the-last-year-demand.”
Due to old software performance, data processing is delayed. The information on inventories is frequently inconsistent because the revenue management function is often separated from the property management system.
The potential of the next-gen RM systems can be realized using existing machine learning and programming techniques
These problems lead to inefficient pricing and timing, which results in low occupancy and significant revenue losses for hoteliers. The modernization of revenue management systems can significantly change the situation. By linking new modules with a property management system, you can prevent overbooking and selling below costs.
How machine learning can improve hospitality revenue management
RM principles remain the same regardless of underlying software. Nevertheless, a tangible quality shift started in the hospitality industry as machine learning and data science-based techniques were introduced in revenue management software.
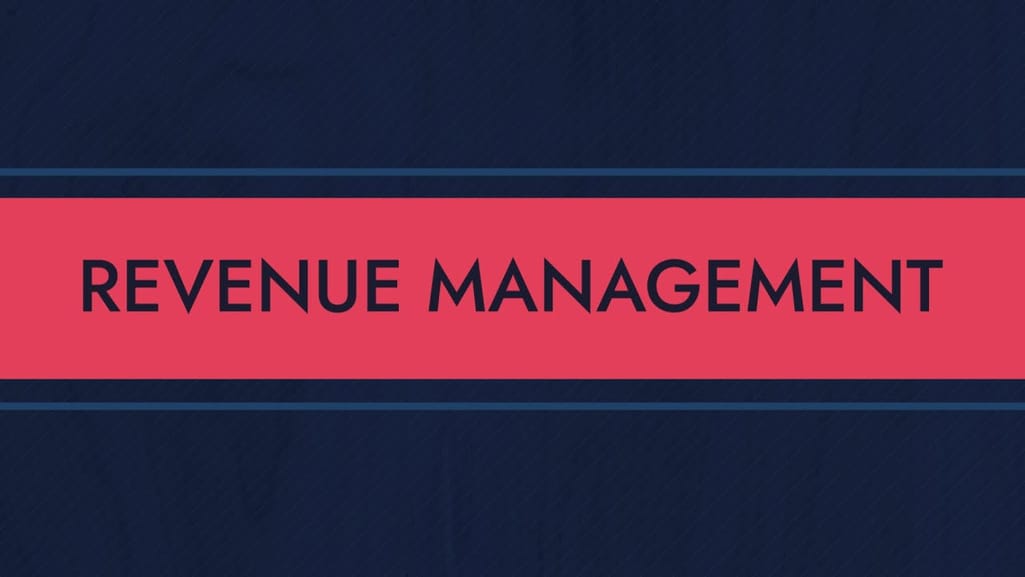
Revenue management in hospitality
Machine learning entails building and training statistical models using data inputs to classify input items or forecast output continuous values. Generally, there are two commonly used types of machine learning implementation: supervised and unsupervised learning. The former requires a historic dataset with labeled output values to base predictions on the new data. The latter uses unlabeled data to find connection between different attributes.
In terms of revenue management, supervised learning techniques allow for predicting best-fit pricing, automatically defining yield tactics, and forecasting demand. Unsupervised learning can be applied to different research tasks such as making highly specified segmentation that accounts for hidden connections between customer demographics and preferences. We’ll discuss practical implementation in more detail below.
These new generation solutions also solve several legacy RM problems suggesting:
- accuracy
- autonomy
- integrity
- real-time data processing.
The RM areas redefined by machine learning applications
Machine learning algorithms influence nearly every component of RM. Let’s have a look at how machine learning based applications drive this change.
While revenue management workflow doesn’t require deep changes, the underlying technology modernization can tangibly increase its efficiency
Segmentation
Segmentation implies dividing guests into groups based on different factors like the purpose of travel, age, marital status, the new or returning customer. As price elasticity differs from customer to customer, precise segmentation impacts price policies as well. Machine learning allows for detailed classification and making tailored offerings to customers based on their needs and price sensitivity.
Another common application is clustering, automatic segmentation based on hidden relations between different data variables. Basically, you can apply clustering algorithms to nuance your segmentation and enrich it with deep customer insights. These may be implicit connections between demographics and price sensitivity or in-hotel behavior and customer lifetime value.
Demand forecasting
Another important part of revenue management is demand forecasting. Machine learning here substitutes such conventional methods as “same-day-last-year,” simple moving average, and linear regression as they don’t account for most of the factors impacting demand and generally lack accuracy.
An ML-based system can analyze market conditions streaming data from travel APIs. So, the algorithm assesses the occupancy of the nearest properties, competitors' prices, public events at the destination, room types, etc. A demand forecast provides critical information for pricing across customer segments and allows for selecting an appropriate distribution strategy. It can also explore what guests want and describe their price sensitivity.
During peak demand periods, hotels strive to maximize revenue; while during low demand periods hotels should maximize room occupancy
Yields
Yield management deals with inventory. In the final analysis, it should optimize profit and revenue, controlling utilization of rooms, conference halls, restaurant, and casino spaces in hotels. Yield management strives to maximize revenue opportunities during high demand days and maximize occupancy during low demand days. This is achieved by quickly covering fixed costs for managing inventories. The technique is particularly important when the proportion of fixed costs is much higher than that of variable costs. The disparity between fixed and variable cost covers overhead and then contributes to profit.
The AI-based system in this case allows for automating the decision-making process in choosing the yield tactics for different customer segments. As the same room can be demanded by different customers, the system should be able to make the best deal applying one or several yield tactics in real time.
Real-time decision making facilitates the ability to rapidly choose the best yield tactic
Pricing
Dynamic pricing is an important part of revenue management. It offers variable room rates based on demand and supply. This allows hotels to quickly react to changing market values maximizing revenue and occupancy rates. Customer segmentation, demand, and supply insights allow hotels to apply different pricing strategies.
Data-driven applications extend the efficiency of pricing strategies. For instance, the ML-based revenue optimization system applied by Starwood Hotels combines different strategies and data sources to come up with the best price for each room at every given moment. As Starwood executives claim, the algorithms behind their dynamic pricing engine account for both the personal profile of a customer, room type and rates, as well as external data, including competitive prices, weather data, and even booking patterns captured on other websites.
Machine learning based revenue management: data sourcing and approaches
All machine learning applications depend on the quality and quantity of data collected from different sources that is then analyzed. In terms of the hospitality industry’s revenue management, the algorithms may include about 700-800 different attributes to build demand, price, and yield management systems. Let’s have a look at different types of data that can be collected and how they can be used to build ML-driven revenue management systems.
Internal data
Internal data can be collected via conventional tracking systems like CRMs or internal booking engines. The key variables in this category are:
- occupancy
- room rates
- bookings dates
- geographical information (where guests are arriving from)
- arrival dates
- departure dates
- revenue by day
- room type
- travel purpose
- inventory cost, etc.
This data can often be easily combined with behavioral data from the website. It’s usually gathered and stored in the CRM and PMS. Two main areas of use for internal data are segmentation and yield optimization.
Segmentation. CRM data contains insights about most loyal guests and allows hotels to better asses price elasticity within segments.
Yield optimization. Real-time information about inventories allows for composing the best allocation of rooms among customers.
Demand forecasting
The market-related information allows for evaluating demand and supply conditions. This usually includes publicly available data:
- competitor rate information
- hotel occupancy at a destination
- flight demand
- online reviews
- canceled reservation
- payment types
- room-level photos
- guest count
- dates of stay.
Additionally, the information from travel APIs provides hoteliers with such details as destination popularity and the scope of competition. Have a closer look at available travel and booking APIs in our comprehensive overview.
Market insights are the main source of information for demand forecasting, as it’s nearly impossible to build accurate prediction models without this information.
External data
External data combines such attributes as weather reports and public events information. The external factors also affect customer willingness to make purchases. For instance, sea temperature might significantly correlate with hotel demand for rooms and conversion probability in multiple customer segments. Compared to single travelers, travelers with children will be more likely to book a long-term stay near a warm body of water.
The common source for this data are such services as OpenWeatherMap API or Picatic API. These APIs can include the following information:
- temperature
- water temperature
- pressure
- wind speed
- event schedules
- event tickets sold number
Public events like sports and festivals create an inflow of tourists. And this data can also be collected using tours and activities APIs from various travel vendors. For instance, Expedia Things to Do API allows for sourcing activities and events info for the nearest 15 days for a chosen location.
Another source of external data is local media outlets. By analyzing the current sentiment, you can estimate tourists’ potential willingness to stay at a given place. So, using external data for demand forecasting and dynamic pricing is critical.
User behavior data
User behavior analysis requires deep understanding of behavior patterns. The main attributes to look at are:
- website traffic values
- referral sources
- time spent on a website
- visit frequency
- clicks
- device type
Behavior data allows for assessing room demand and price sensitivity. So, it’s useful for segmentation of customers who interact with a website, especially those who didn’t log in or visit a website for the first time.
Social media data
User-generated content in social media also provides valuable information on customer preferences. This group of data sources includes tweets, posts, likes, geotags, photos, and text sentiments. Social media is widely used for predicting travelers’ preferences and evaluating their interests. Social media data also helps determine destinations that are just gaining popularity, making tweets, posts, and geotags good variables for demand forecasting.
Final word
Revenue management is a critical function in the hospitality industry. Although the general idea appeared over 50 years ago, hotels are only in early days of its utilization.
Ninety-six percent of hoteliers claim that historical data is the most important success factor in revenue management systems. The implementation of a revenue management system provides properties with a wide range of improvements like increased profitability, maximized occupancy, and reduced time expenditures associated with traditional pricing and reporting. Small and medium size properties are especially inefficient at revenue generation and profits management. Implementation of data-driven pricing software allows for increasing return on investment (ROI) by 5-10 percent. Revenue per available room increases by 7.5-10 percent on average. The approach demonstrates high results with 97 percent of hotels claiming sales growth after implementation.
Machine learning algorithms enhance the efficiency of revenue management systems. Real-time data processing, personalization, and automation are the main traits that allow for outperforming the legacy systems in determining price and time to make a satisfactory offer and maximize revenue. A good practice is to engage external data science experts to build segmentation and forecasting features. Such solutions require gathering user information, which is difficult to do without a custom-built behavior and market tracking engine.