In this article, we’ll highlight 5 use cases of AI-based technology adoption in manufacturing. We will also address the success stories of modern manufacturing companies as we analyze how implementation of AI benefited their businesses.
Predictive Maintenance to Forecast Remaining Useful Life of Equipment
Predictive maintenance is a strategy that entails continuous monitoring of equipment’s state under normal working conditions and predicting remaining useful life. While reactive and preventive maintenance help decrease or just prevent failures, predictive maintenance uses models to forecast when a specific asset is about to have a component fail. This minimizes downtime and helps schedule maintenance in advance.Speaking about manufacturing, we should consider the high cost of suspending production especially dealing with big enterprises. With predictive maintenance, there is no need to suspend your manufacturing processes as it helps detect even those minor changes in equipment’s state that are not detectable with a typical inspection. AI-based diagnostic tools enable manufacturers to determine circumstances that may cause breakage and intervene before it happens. Using machine learning models, manufacturers can predict the remaining useful life of equipment and prepare it for further repair.
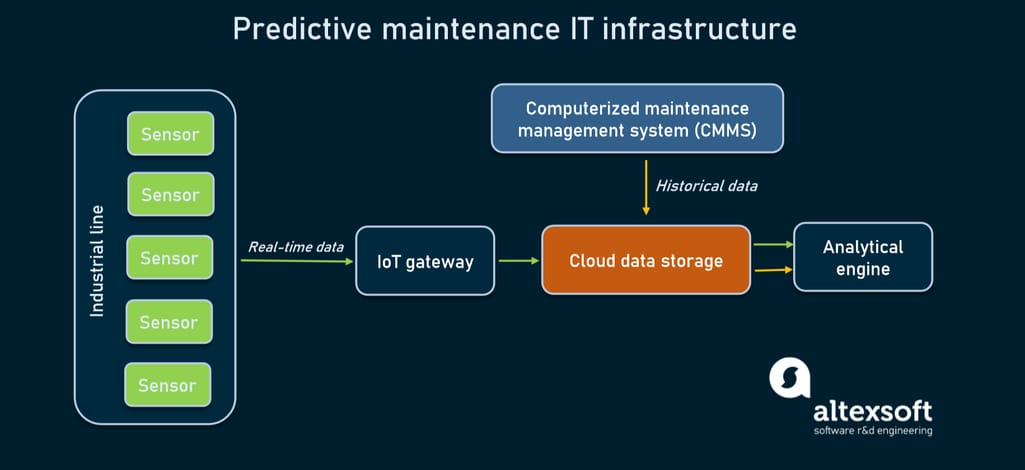
Main hardware and software components powering predictive maintenance
Let’s talk about the Dutch railway company and the international player in the sphere of railway maintenance, Strukton Rail. Managing 40 percent of the Dutch railway system, the company was challenged to find the right model to effectively predict rail switch failures to make the overall maintenance process monitored and well-planned without affecting daily traffic. Switch failure comprises the biggest category of failure causes and solving this problem would greatly improve the overall network and infrastructure performance. According to Strukton Rail, predictive maintenance as a solution to this problem leads to higher rail availability at lower costs.Some of the reasons for switch failure included electrical problems (worn-out brushes, motor), lack of grease on slide chairs, bent blades due to weather conditions, poor adjustment of rolling constructions, and so on.
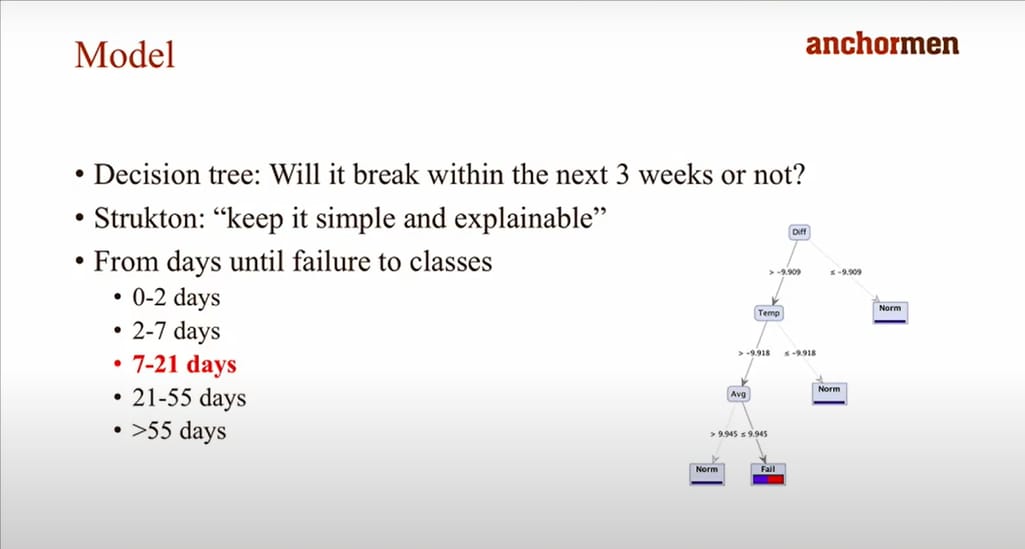
Here is the decision-tree model chosen for predicting rail switch failure, Image source: Anchormen
How does it work? Strukton’s predictive maintenance monitoring system includes hardware that consists of data loggers and non-intrusive sensors. About half of the 3,000 points that Strukton Rail manages in the Netherlands are equipped with sensors that record data about the energy consumption of the points motors when turning the points. This data comes from different sources (such as point heating, rail temperature, level crossing, etc.) and is further sent to the in-house developed monitoring system POSS (which is a Dutch abbreviation for Structon Preventive Maintenance and Fault Diagnosis System) via the internet. The GPS data and non-conformance alerts are combined for further analysis. As a result, railway operators can timely intervene when it’s needed.Strukton Rail reported that predictive maintenance made it possible to halve the number of technical failures. The company is going to expand POSS with a forecasting tool to predict impending failures and such application of AI-based predictive maintenance can be suitable not only for the Dutch railway, but others as well.
Robotic Process Automation to Streamline Paperwork
In manufacturing, process automation is responsible for using production management software or robotic machines to automate complex processes. While modern manufacturing is often associated with physical robots, it is not the only case as a lot of manufacturing processes also use various management software systems such as, for instance, RPA.Robotic Process Automation (RPA) uses software AI-based technologies and machine learning capabilities to handle high volume repetitive tasks that previously required a human workforce. These tasks can include maintenance of records, addressing queries, making calculations, and so on. The work of RPA includes three main steps: training, operation, and orchestration. During the training phase, a machine has to receive certain instructions for performing the required tasks. Operation is the phase when the bot does what it’s trained for, while the orchestration step is required only when there are multiple bots for performing a range of tasks.
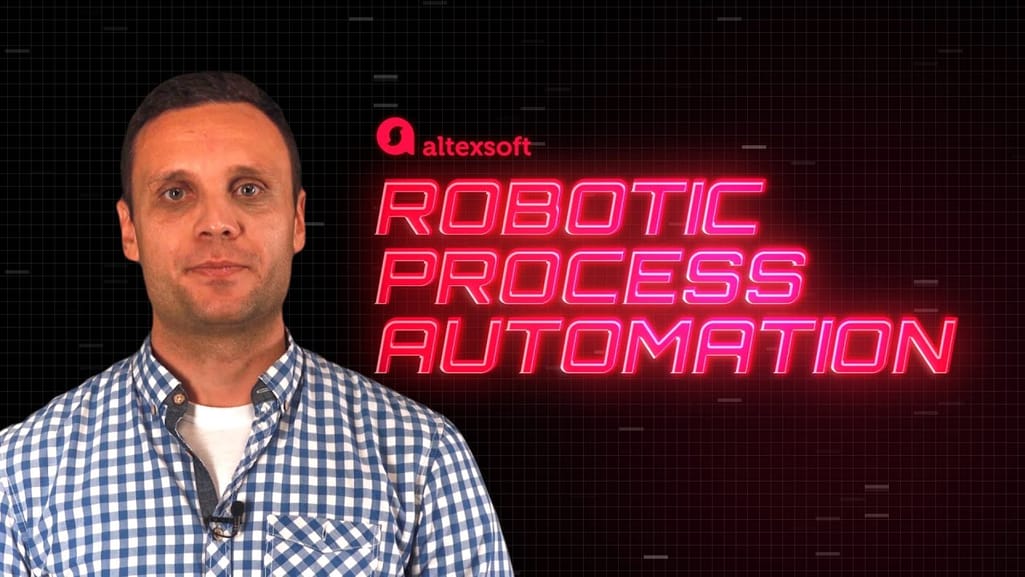
RPA explained in 10 minutes or less
French multinational company in digital automation and energy management Schneider Electric decided to go for RPA to eliminate tasks that had no added value (such as automating document preparation etc.) As a result, the company managed to save some time for their employers to refocus them on activities geared towards customer satisfaction.The problem was that operators responsible for installing electrical switchboards at client premises had to deal with tedious and extensive paperwork such as adding labels identifying electrical circuits or manufacturing specifications of switchboards. To handle this time-consuming and exhausting task, an AI-based bot was introduced to free up operators for more valuable and complex manufacturing-undertakings. A robot developed in just two and a half days successfully completed this task, opening and printing documentation as it was required, freeing up the operators.
Quality Assurance & Inspection of a Manufactured Product with Computer Vision
Quality Assurance in manufacturing relates to the processes applied to maintain consistent quality levels. QA in manufacturing has great potential for applying AI-based computer vision systems to automate inspection throughout the entire production process. Human vision has its obvious cons such as fatigue and inconsistency, but with computer vision inspection, QA becomes more reliable and precise.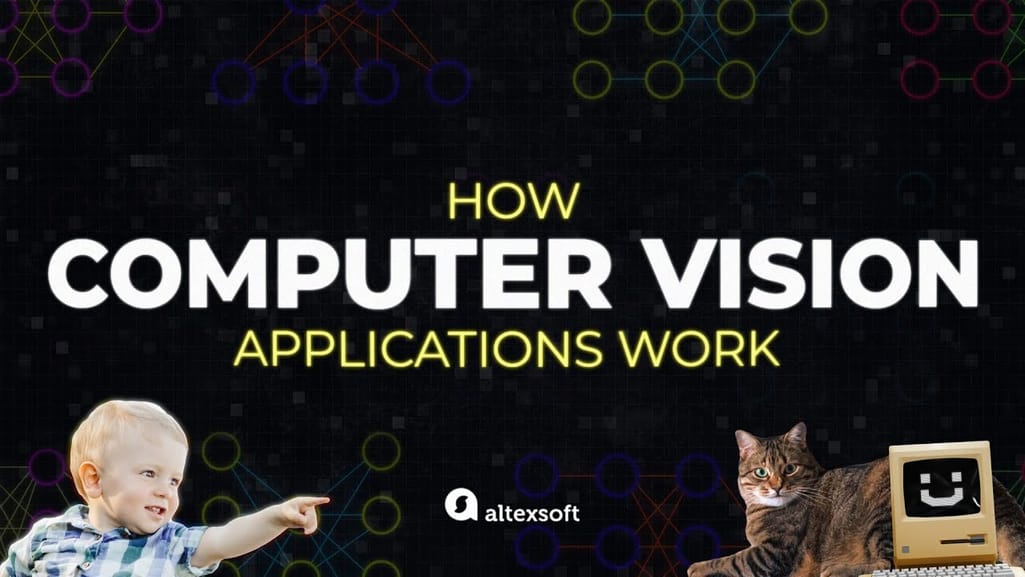
More insight on how computer vision applications work
Foxconn, manufacturing electronic products for such giants as Apple, Nintendo, Nokia, Sony, and others, successfully adopted Google Cloud Visual Inspection AI for quality control in its factories. This machine learning program launched by Google in 2021 helps manufacturers inspect product defects, and, eventually, decrease costs of QA.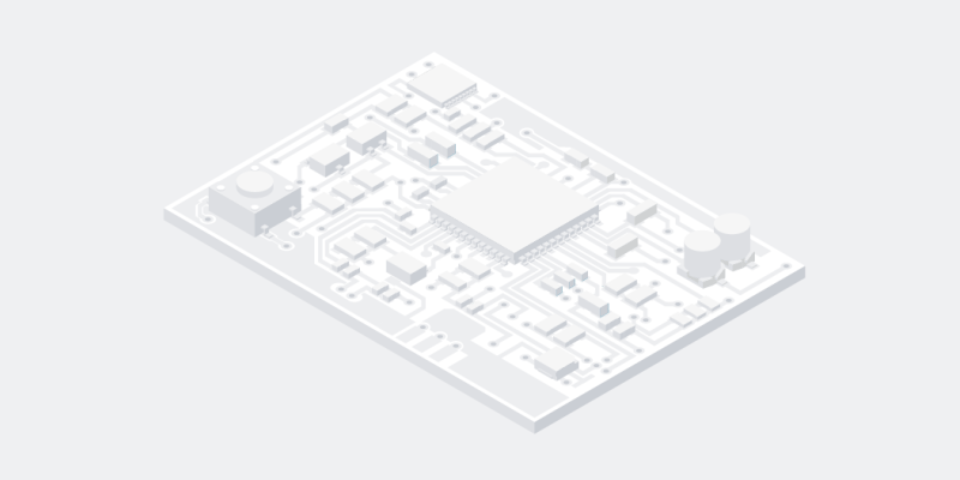
Visual Inspection AI automates QA workflow and identifies defects before products leave the assembly line, Image source: Google
Let’s look at an example of producing smartphones. The final quality of the device directly depends on detecting defects on a PCBs (Printed Circuit Board) during the pre-production phase of manufacturing. PCB can have multiple assembly defects like missing screws or solder bridging. Google’s visual inspection reduced the defect escape rate to 10 percent. Moreover, it decreased inspection time for every component to 0.3 seconds.Belgian developer and supplier of fiberglass products and technologies in thermoplastic and thermoset polymers reinforcement, 3B-Fiberglass, decided to adopt AI-based video inspection after coming across a challenge of fiberglass breaks during one of the production stages. An operator should be informed about the potential break in advance to quickly fix the problem before it affects the further manufacturing process.
3B defined two directions to solve this problem. First, they wanted to identify a reason for a break. Second, they aimed to predict when a break was going to happen and halt the process.
How was AI implemented in this case?

This is how fiberglass production looks schematically, Image Source: CompositesWorld
The fiberglass manufacturing process consists of hot and cold phases. During the former, a set of specific additives are melted and heated up in a furnace to a temperature sufficient for creating glass. Then comes the cold phase where already molten glass is channeled to the special metal alloy plates called bushings. To become fibered, glass has to pass through holes in bushings (with an average diameter of about 10-25 micrometers). The break of a fiber occurred when the glass was running through these holes and the pulling force was applied to it. The company set up a camera that uninterruptedly monitored fibers as they left a bushing. Afterwards, the machine learning network analyzed the received data and predicted the moment of a break. Three-year data was collected and analyzed from channels inside the furnace and close to the panels.
Break of a single fiber on a bushing inevitably leads to stopping the entire flow, Image Source: CompositesWorld
With the help of AI computer-vision analysis, 3B-Fiberglass managed to predict a fiber break approximately 75 seconds in advance, as it was initially planned. Still, the specific cause of the break remained unknown. Most probably, it relates to the heterogeneous nature of the analyzed data as at 3B data is often generated for the monitoring of the overall process which complicates the root-cause analysis of a particular break.Demand Forecasting to Improve Supply Chain Efficiency
Demand Forecasting in manufacturing is a process aimed at predicting future demand for a specific product. Accurate demand forecasting helps manufacturers reduce risk and increase overall supply chain efficiency. However, this term should not be confused with demand planning as the latter is a broader concept that includes demand forecasting, but doesn't consist entirely of it.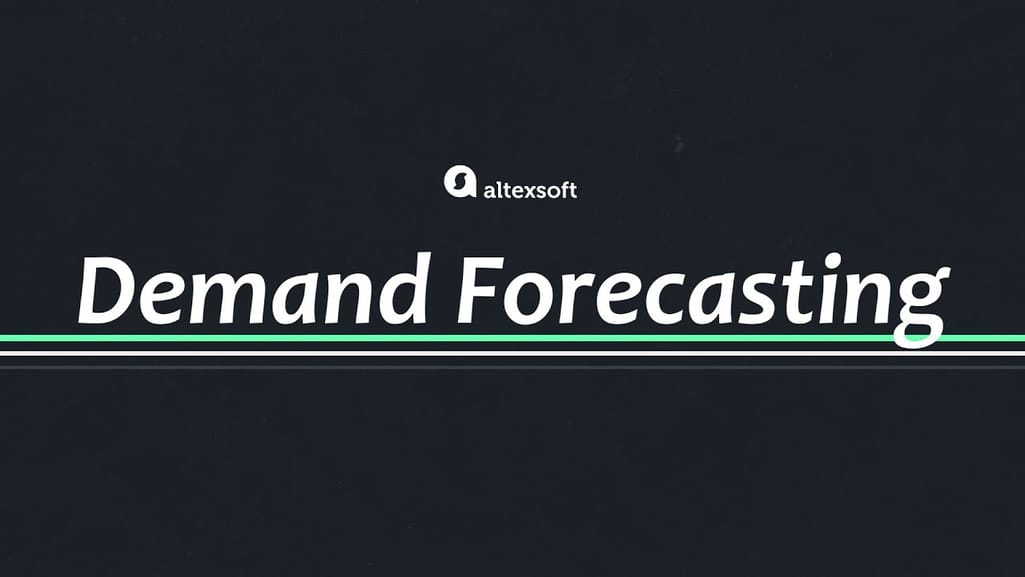
Demand forecasting explained
Machine learning algorithms can be used to successfully predict consumer demand as well as any changes related to it. Recognizing recurring patterns and complex relationships, machine learning systems process historical sales and supply chain data, and analyze thousands of factors that drive buying behavior. Unlike traditional forecasting, ML forecasting can work with large amounts of data. Consequently, it can be a solution for both short-term and mid-term planning of new products. Even though it is impossible to achieve 100 percent accuracy, AI-powered demand forecasting benefits manufacturers by improving workforce and financial planning.In 2011, French multinational manufacturer of food products Danone implemented an ML system to create a more accurate demand forecasting platform. For a partner, the manufacturer chose a global provider of supply chain optimization software systems ToolsGroup. The forecasting process addressed a variety of fresh products united by such factors as short shelf life and dynamic demand. Capturing both sales and demand stories of these products, the provider defined insufficient stock periods and analyzed how to fix these issues in future demand planning.
As a result of ML demand forecasting, Danone managed to reduce forecast errors by 20 percent and lost sales by 30 percent. What's more, company demand planners got more free time to refocus on activities with higher added-value.
Warehouse Automation to Improve Interoperability of Vehicles
For manufacturers, warehouse automation becomes a relevant solution to minimize manual labor and reduce operational costs. Automated warehousing also helps companies process orders quicker and ensures more accurate scheduling.In 2018, internationally known car manufacturer Toyota presented its AI Team Logistics concept at the CeMAT exhibition. The concept proposes to modernize how horizontal transporters can communicate with AI-based, high-level machinery in real time. The main idea is to optimize all truck movements in the warehouse and make them interconnected.
Every second the AI software system calculates the optimal use of resources and route for the transporters. Such direct communication between vehicles replaces the traditional central warehouse concept by machine teamwork. Maintaining fully automated continuous flow, the company aims to optimize warehouse processes.

AI Team Logistics concept transforms superfluous warehouse management into a productive teamwork, Image Source: Toyota
Challenges and Pitfalls of AI Adoption
Here are some challenges a manufacturer can face when adopting AI-based technologies:High cost of AI adoption. The initial implementation of AI can be expensive, especially for small companies and startups taking into account data acquisition and engineering costs. However, a lot depends on the problem a manufacturer wants to solve with the help of artificial intelligence. If the in-house team needs to develop AI systems ab initio, the price will get higher than for consulting a narrow-specialized expert.
By the way, we have an article about measuring ROI in machine learning. Give it a look!
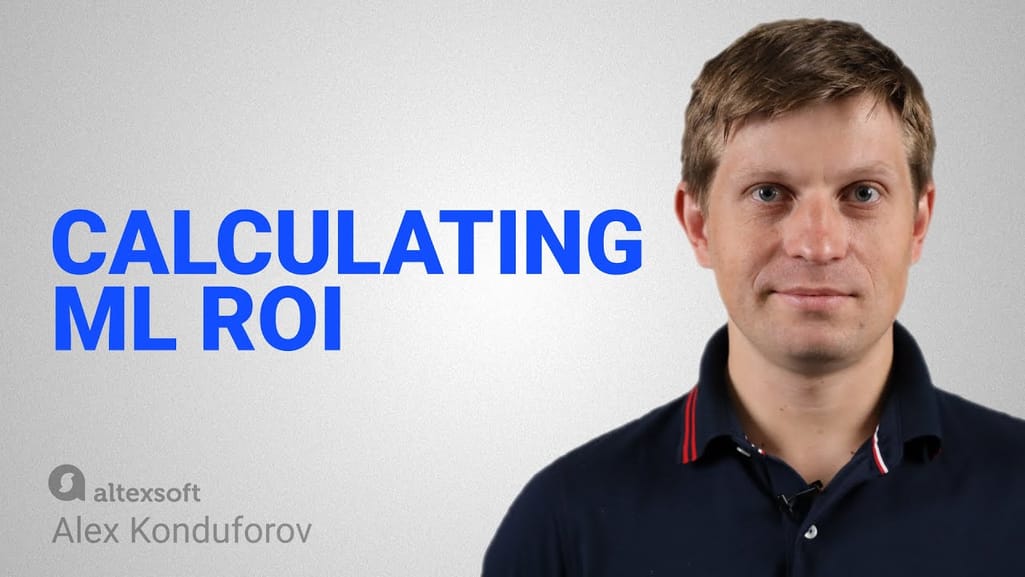
How to justify ML investments
The lack of skilled experts. Even though AI presupposes the surge in robotic automation systems, machine learning technologies are constantly evolving. If there are enough skillful data scientists on your in-house team, good for you. Otherwise, you will need to look for AI professionals with relevant experience.Data Quality. In manufacturing, data often appears outdated, biased or fallible, but the overall success of AI adoption directly depends on the quality of the data. For instance, if we speak about plants, here data is frequently built on unconnected systems patented exclusively as a property of a manufacturer. This makes it not always applicable for broad AI-based analytics.
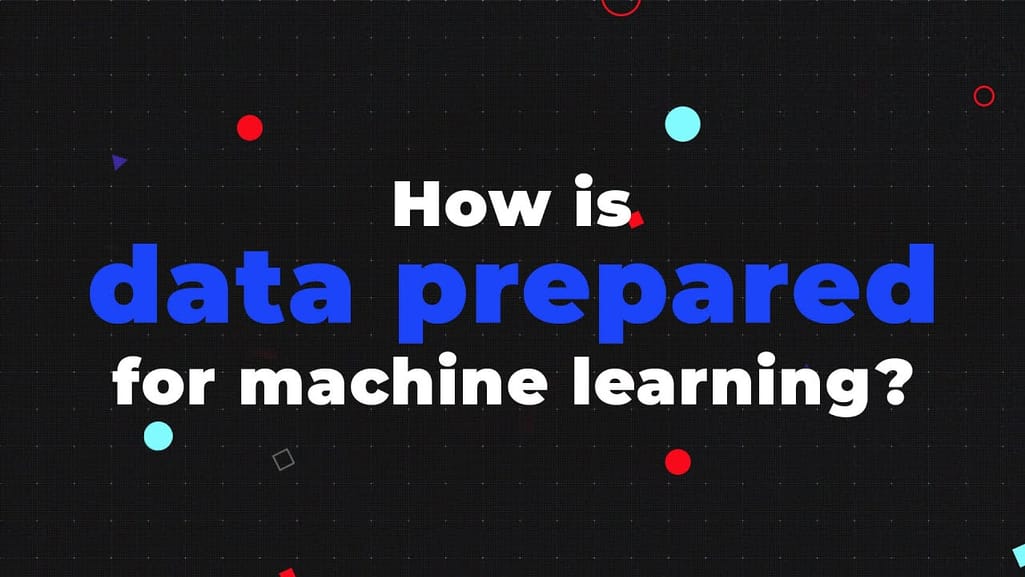
Learn more about data preparation for ML
AI and the Future of Manufacturing: What to Wait for?
We are living in the Fourth Industrial Revolution (also known as Industry 4.0), marking the rapid transformation of all manufacturing processes. Implementing AI-based technologies has inevitably changed the way goods and services are planned and produced today.Probably, the best word to define Industry 4.0 would be digitalization as here resources, information, and humans are connected with the help of modern digital systems. As we know, traditionally, manufacturing was an area that trained humans to think like machines. However, with the advent of digitalization, the coin flipped and now machines are quite successfully trained to think like humans.
Among the technologies widely associated with Industry 4.0, are Smart Factory, Smart Products, Robots, Simulation, etc. For sure, the best is yet to come and we will see progressive changes in manufacturing very soon.
So, here are some powerful trends that are already implemented in practice and will certainly revolutionize the entire manufacturing industry.
Digital Twins
A digital twin is a digital representation of a physical product in all its aspects. This copy covers the whole lifecycle of its physical counterpart. So, digital twins are able to simulate any physical object or process, allowing engineers to digitally create and maintain complex products, machines, factories, or even entire supply chains.Digital twins can be applied for various use cases:
- Predictive Maintenance
- Remote Troubleshooting
- Product / Performance Optimization
Internet of Things (IoT)
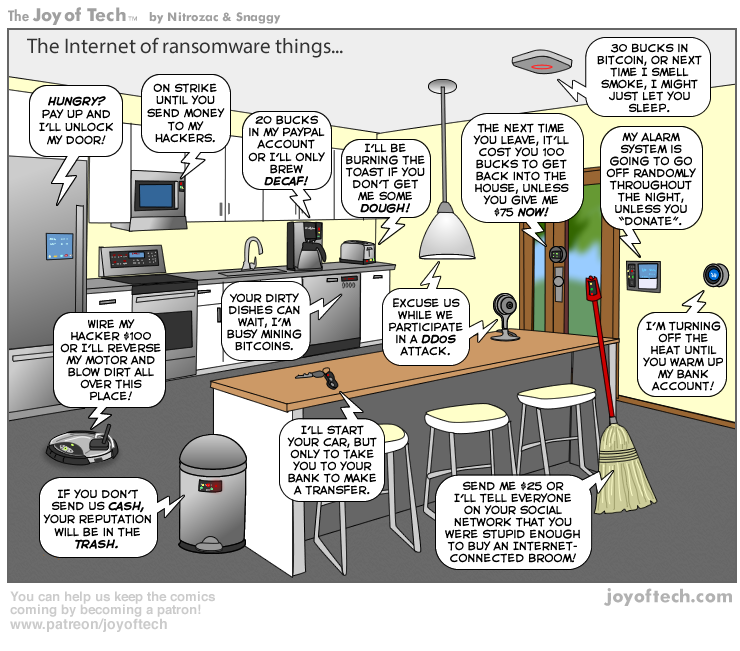
Let’s all agree that “Measure is a treasure”, Image Source: Redelijk Heid
Internet of Things is a term used to describe a dynamic global network of connected physical objects embedded with software, sensors, or other technologies exchanging data with other devices. The basic elements of IoT include a central control hardware, multiple connected devices, data cloud, and user interface.A perfect example of both Digital Twins and the Internet of Things would be Microsoft’s launch of Azure Digital Twins. This IoT platform helps create a digital representation of manufacturing - and not only - processes and enables the optimization of costs and operations. Azure Digital Twins can help you as a manufacturer define your business environment by defining the custom twin types (usually referred to as models).
The new era will be the time of smart connected machines where humans complement their working environment with intelligent cobots. Here, the task of modern manufacturers is to make sure they are well-prepared for the newest digitalization and know how to shape their business process to leverage all these new technologies.